Single-piece job shop scheduling method based on Deep Q-network deep reinforcement learning
A job shop, reinforcement learning technology, applied in neural learning methods, machine learning, biological neural network models, etc.
- Summary
- Abstract
- Description
- Claims
- Application Information
AI Technical Summary
Problems solved by technology
Method used
Image
Examples
Embodiment Construction
[0059] The present invention will be described in more detail below in conjunction with the accompanying drawings and through specific embodiments.
[0060] The present invention is a job shop scheduling method based on Deep Q-network (DQN) deep reinforcement learning, and its flow is as follows figure 1 shown, including the following steps:
[0061] (1) Taking the ft06 benchmark case as the specific implementation description, and taking the minimum and maximum completion time as the performance evaluation index, the information of the ft06 benchmark case is shown in Table 1, and the disjunctive graph is used to establish the scheduling environment of the benchmark case, as shown in figure 2 shown;
[0062] Table 1 ft06 benchmark example
[0063]
[0064] (2) Extract state features from the disjunctive graph as the input of the convolutional neural network;
[0065] The principle of state design: From the perspective of task goals, the selection of state features shoul...
PUM
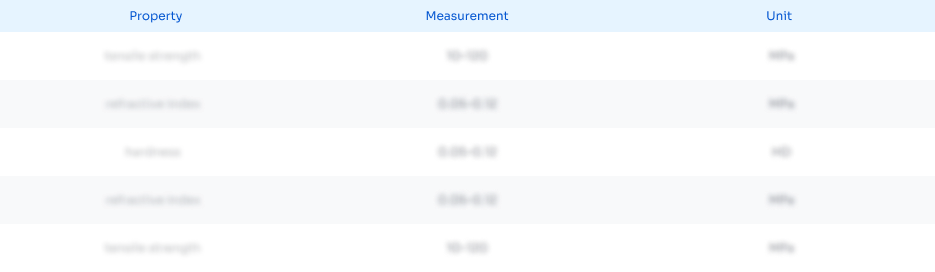
Abstract
Description
Claims
Application Information
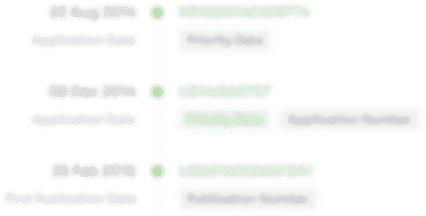
- R&D
- Intellectual Property
- Life Sciences
- Materials
- Tech Scout
- Unparalleled Data Quality
- Higher Quality Content
- 60% Fewer Hallucinations
Browse by: Latest US Patents, China's latest patents, Technical Efficacy Thesaurus, Application Domain, Technology Topic, Popular Technical Reports.
© 2025 PatSnap. All rights reserved.Legal|Privacy policy|Modern Slavery Act Transparency Statement|Sitemap|About US| Contact US: help@patsnap.com