Liver tumor MVI prediction method based on triple network
A technology of liver tumor and prediction method, applied in the field of MVI prediction of liver tumor based on triplet network, can solve the problem of fine-grained image features, constraints, image feature definition and extraction without considering the similarity of MRI images of HCC patients. Great impact and other problems, to achieve the effect of good classification and recognition ability, expansion of distance, and reduction of distance
- Summary
- Abstract
- Description
- Claims
- Application Information
AI Technical Summary
Problems solved by technology
Method used
Image
Examples
Embodiment Construction
[0036] In order to make the purpose, technical solutions and advantages of the present invention clearer, the technical solutions in the embodiments of the present invention will be clearly described below in conjunction with the accompanying drawings in the embodiments of the present invention. Obviously, the described embodiments are part of the present invention Examples, not all examples. Based on the embodiments of the present invention, all other embodiments obtained by persons of ordinary skill in the art without making creative efforts belong to the protection scope of the present invention.
[0037] combine Figure 1 to Figure 4 As shown, the embodiment of the present invention provides a method for predicting liver tumor MVI based on triplet network, such as figure 1 shown, including the following steps:
[0038] S101: Divide the patient's liver MRI image data into a training set and a test set; select three samples from the training set to form a triplet sample; ...
PUM
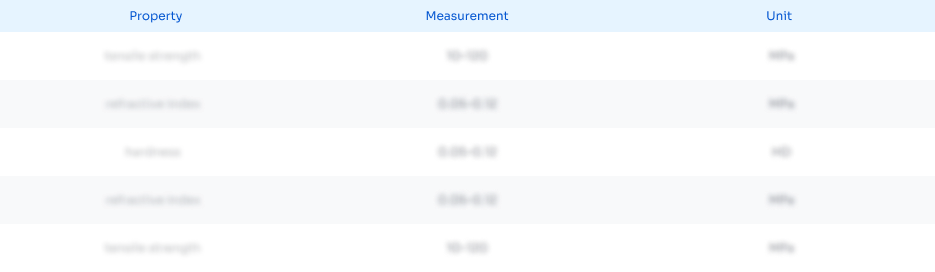
Abstract
Description
Claims
Application Information
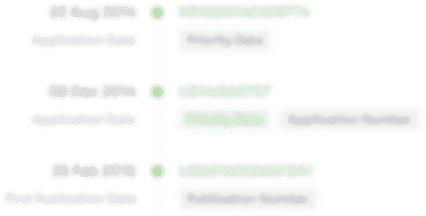
- R&D Engineer
- R&D Manager
- IP Professional
- Industry Leading Data Capabilities
- Powerful AI technology
- Patent DNA Extraction
Browse by: Latest US Patents, China's latest patents, Technical Efficacy Thesaurus, Application Domain, Technology Topic, Popular Technical Reports.
© 2024 PatSnap. All rights reserved.Legal|Privacy policy|Modern Slavery Act Transparency Statement|Sitemap|About US| Contact US: help@patsnap.com