Industrial part surface defect detection method and system based on anomaly detection algorithm
An anomaly detection and defect detection technology, applied in the field of deep learning anomaly detection, can solve the problems of difficult collection of low-frequency samples, long data collection cycle, and high labor costs, so as to save model training and data collection time, and promote projects as soon as possible and acceptance , The effect of tight production beat
Inactive Publication Date: 2022-01-07
菲特(天津)检测技术有限公司
View PDF6 Cites 1 Cited by
- Summary
- Abstract
- Description
- Claims
- Application Information
AI Technical Summary
Problems solved by technology
However, this method has the following defects: Low efficiency: the efficiency of inspecting parts actually tests a person's proficiency. Longer working hours will have higher detection efficiency, but as working hours increase, personal fatigue and laziness will also increase. increase, which will reduce the detection efficiency of quality inspectors; risk of missed inspection: as the working hours increase, personal attention will also decrease, which will bring the risk of missed inspection, and the machine will not be tired, so there is no such problem; it is difficult to define : Since the size of industrial defects is at the millimeter level, it is difficult to manually identify millimeter-sized defects with the naked eye; quantitative analysis is difficult: manual determination of defects will not be able to carry out data statistics, which will hinder the intelligentization of factories; high labor costs : Enterprises will continue to pay corresponding expenses for manual quality inspection with high uncertainty, and the cost-effectiveness is low; further adopting traditional visual algorithms, the writing of artificial non-standardized algorithms has great limitations, and only by judging the gray value or area Defect judgment will increase the risk of misjudgment; and the algorithm writing process is complicated and the versatility is not high. If there are many models, each model will cost algorithm engineers to write algorithms, and the labor cost is high.
[0006] (1) Defect unknown: only known defect categories can be continuously added. If unknown defect categories appear in the future, the equipment will lose its proper function and bring immeasurable losses to the manufacturer.
[0007] (2) Difficulty in defect collection: It is difficult to collect defect data sets, man-made or synthetic defects are greatly different from real defects, there are low-quality sample data, and the data collection cycle is long
[0008] (3) Difficulty in intercepting low-frequency defects: even for defects with known and sufficient data sets, there will be defects with characteristics that are not similar to this type, and there may be missed detections
[0009] The difficulty of solving the above problems and defects is: due to the limitation of the target detection range, it will be difficult for the device to intercept defects of unknown categories in known data sets, or subcategories of existing defects
Low-frequency samples are difficult to collect, and it is difficult for algorithms to detect them
Method used
the structure of the environmentally friendly knitted fabric provided by the present invention; figure 2 Flow chart of the yarn wrapping machine for environmentally friendly knitted fabrics and storage devices; image 3 Is the parameter map of the yarn covering machine
View moreImage
Smart Image Click on the blue labels to locate them in the text.
Smart ImageViewing Examples
Examples
Experimental program
Comparison scheme
Effect test
Embodiment
[0087] Step 1. Collect data for the model to be inspected. After the parts enter the equipment, they go to the abnormality detection station, and take pictures of the parts with a fixed camera to ensure that the image area of the parts obtained after shooting is fixed and has similar characteristics.
the structure of the environmentally friendly knitted fabric provided by the present invention; figure 2 Flow chart of the yarn wrapping machine for environmentally friendly knitted fabrics and storage devices; image 3 Is the parameter map of the yarn covering machine
Login to View More PUM
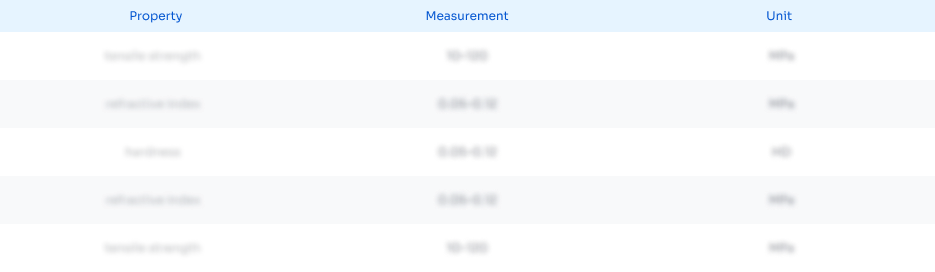
Abstract
The invention belongs to the technical field of deep learning anomaly detection, and discloses an industrial part surface defect detection method and system based on an anomaly detection algorithm. The method comprises the following steps: obtaining an image of a to-be-detected product, and collecting the surface image data of a to-be-detected good product shot by an industrial camera; the image is input into a teach-student anomaly detection network model to be trained; detecting defect data and non-defective product data by using a model produced after training is completed; returning a detection result to the client, and displaying the detection result by the client; and transmitting the detected abnormal region and the size of the region to a client of software, and judging whether the defect needs to be eliminated or not through an area threshold value on the software. The detection of surface abnormal areas of most parts with simple surface structures can be met. Compared with target detection, a large amount of model training and data collection time is saved, and the method has a great promotion effect on project promotion and acceptance as soon as possible.
Description
technical field [0001] The invention belongs to the technical field of deep learning anomaly detection, and in particular relates to a method and system for detecting defects on the surface of industrial parts based on a deep learning anomaly detection algorithm. Background technique [0002] At present, in the field of industrial defect detection, the defect recognition technology using deep learning target detection has become mature, but the disadvantages of target detection have also emerged. In the process, a large amount of defect data needs to be provided for the neural network to learn, but because the defect imaging has influence factors such as position, shape, light source, etc., different factors will combine to form a variety of defects, and there may be very few and large shapes. The unknown defects will make it extremely difficult for AI target detection algorithms to learn. Moreover, it is difficult to collect real defects of industrial parts, which may cont...
Claims
the structure of the environmentally friendly knitted fabric provided by the present invention; figure 2 Flow chart of the yarn wrapping machine for environmentally friendly knitted fabrics and storage devices; image 3 Is the parameter map of the yarn covering machine
Login to View More Application Information
Patent Timeline
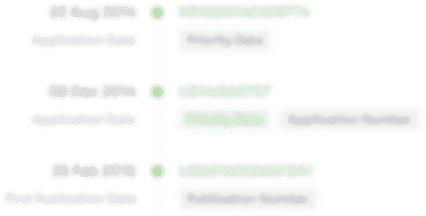
Patent Type & Authority Applications(China)
IPC IPC(8): G06T7/00G06T7/62G06V10/774G06K9/62G06N3/04
CPCG06T7/0004G06T7/62G06N3/045G06F18/214
Inventor 王凯陈立名田楷晏文仲黄金张健浩杨剑远
Owner 菲特(天津)检测技术有限公司
Features
- R&D
- Intellectual Property
- Life Sciences
- Materials
- Tech Scout
Why Patsnap Eureka
- Unparalleled Data Quality
- Higher Quality Content
- 60% Fewer Hallucinations
Social media
Patsnap Eureka Blog
Learn More Browse by: Latest US Patents, China's latest patents, Technical Efficacy Thesaurus, Application Domain, Technology Topic, Popular Technical Reports.
© 2025 PatSnap. All rights reserved.Legal|Privacy policy|Modern Slavery Act Transparency Statement|Sitemap|About US| Contact US: help@patsnap.com