Cold-chain logistics temperature prediction method, and temperature regulation and control method
A technology of cold chain logistics and forecasting methods, which is applied in the direction of temperature control using electric methods, thermometers, thermometer applications, etc., and can solve problems such as incomplete consideration of factors, failure to consider the influence of spatial location temperature, and slow convergence speed of neural networks. , to achieve the effect of avoiding too low temperature regulation, improving prediction accuracy, and good global search ability
- Summary
- Abstract
- Description
- Claims
- Application Information
AI Technical Summary
Problems solved by technology
Method used
Image
Examples
Embodiment Construction
[0037] Specific embodiments of the present invention will be described in detail below in conjunction with the accompanying drawings.
[0038] In order to understand the method better, the extreme learning machine and the ephemera algorithm related to the present invention are introduced first.
[0039] 1. Extreme learning machine
[0040] The extreme learning machine is an advanced single hidden layer feedforward neural network algorithm, which overcomes the situation that the result is easy to fall into local optimum and slow convergence speed due to the use of gradient descent algorithm in the traditional feedforward neural network. The extreme learning machine is composed of input layer, hidden layer and output layer, and its network structure is as follows: figure 1 shown. Use the extreme learning machine to train N arbitrary samples (x i ,y i ), where x i represents the input, y i represents the corresponding x i The expected output of the extreme learning machine...
PUM
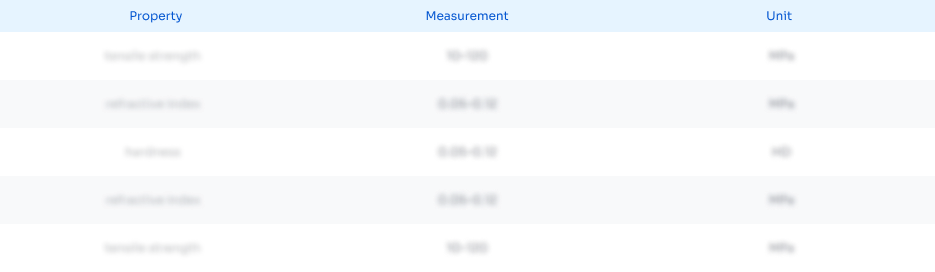
Abstract
Description
Claims
Application Information
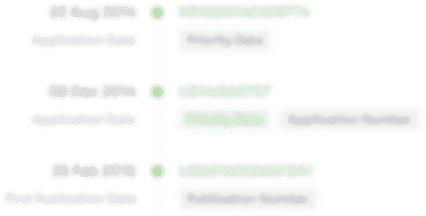
- R&D Engineer
- R&D Manager
- IP Professional
- Industry Leading Data Capabilities
- Powerful AI technology
- Patent DNA Extraction
Browse by: Latest US Patents, China's latest patents, Technical Efficacy Thesaurus, Application Domain, Technology Topic, Popular Technical Reports.
© 2024 PatSnap. All rights reserved.Legal|Privacy policy|Modern Slavery Act Transparency Statement|Sitemap|About US| Contact US: help@patsnap.com