Underground structure internal defect identification method based on cross-hole radar and deep learning
A technology of deep learning and underground structure, applied in neural learning methods, character and pattern recognition, neural architecture, etc., can solve the problem of high computational cost of full waveform inversion method, high requirements for parameter selection, and tomographic imaging method Problems such as low resolution, to achieve the effect of ensuring generalization ability, high resolution and fast recognition, and resolution enhancement
- Summary
- Abstract
- Description
- Claims
- Application Information
AI Technical Summary
Problems solved by technology
Method used
Image
Examples
Embodiment 1
[0042] Such as figure 1 as shown, figure 1 It is a schematic flowchart of a method for identifying internal defects in underground structures based on cross-hole radar and deep learning provided by an embodiment of the present invention. Its schematic defect identification method includes the following steps:
[0043] Step S1: establish a numerical simulation data set, the numerical simulation data set includes several groups of data pairs of cross-hole radar time-domain waveform diagrams and model dielectric constant distribution diagrams;
[0044] Exemplarily, taking the internal defect detection of an underground diaphragm wall as an example, the step S1 may include:
[0045] Step S101: Establish a three-dimensional numerical simulation dielectric constant model of the underground diaphragm wall, such as figure 2 shown. The model contains background medium and defects, and the type, position, size and filling medium of defects in any single model are randomly selected....
PUM
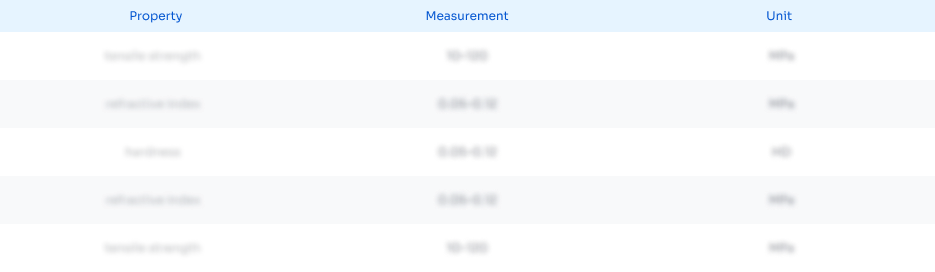
Abstract
Description
Claims
Application Information
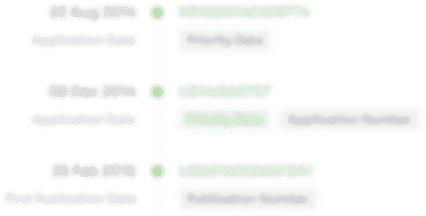
- R&D
- Intellectual Property
- Life Sciences
- Materials
- Tech Scout
- Unparalleled Data Quality
- Higher Quality Content
- 60% Fewer Hallucinations
Browse by: Latest US Patents, China's latest patents, Technical Efficacy Thesaurus, Application Domain, Technology Topic, Popular Technical Reports.
© 2025 PatSnap. All rights reserved.Legal|Privacy policy|Modern Slavery Act Transparency Statement|Sitemap|About US| Contact US: help@patsnap.com