Image enhancement method and device for diabetic renal lesion classification
A kidney lesion and image enhancement technology, applied in the field of medical image processing, can solve problems such as low quality of CT images and limit the classification accuracy of image classification models, and achieve the effects of improving image quality, feature extraction and fusion, and improving efficiency
- Summary
- Abstract
- Description
- Claims
- Application Information
AI Technical Summary
Problems solved by technology
Method used
Image
Examples
Embodiment 1
[0050] The super-resolution reconstruction network architecture and the structure of each module in the present invention are as follows Figure 1-Figure 6 As shown, the MDC feature extraction unit 2 is set to eight, the preliminary feature extraction unit 1 is a 3*3 convolutional layer, and the second image 6 input to the super-resolution network is a three-channel image. After the preliminary feature extraction unit 1, Obtain the first feature map with a channel number of 48. Inside the MDC feature extraction unit 2, the number of G1, G2, and G3 feature map channels extracted by the three branches is 48. After G1 and G2 undergo element summation, 1*1 convolution, and ReLU activation function, they are combined with G2, G2, and G3. G3 splicing to obtain the G4 feature map with 144 channels.
[0051] Inside the enhanced channel attention module 22, such as Figure 4 As shown, the four branches are in a parallel relationship, and the four branches all receive the G4 feature m...
Embodiment 2
[0057] As a comparative experiment, in the case that the relevant factors such as the control data set and the loss function are exactly the same, only the enhanced channel attention module 22, the modulation module 4 and its connection structure of the super-resolution reconstruction network provided by the present invention in Example 1 are used modified as Figure 7 Shown as Comparative Example I. In Comparative Example I, the four branches modulated the feature map G4 and spliced at the same time. The enhanced channel attention module 22, modulation module 4 and its connection structure of the super-resolution reconstruction network provided by the present invention in embodiment 1 are modified as follows Figure 8 Shown as Comparative Example II. In Comparative Example II, the third branch 25 in the enhanced channel attention module 22 is removed.
[0058] Using exactly the same data set as in Example 1, after the training of Comparative Example I and Comparative Exa...
PUM
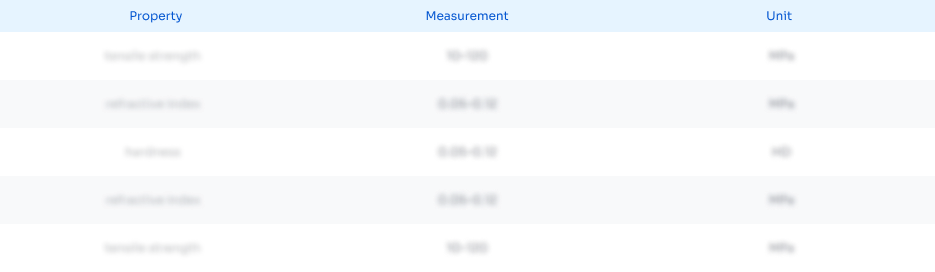
Abstract
Description
Claims
Application Information
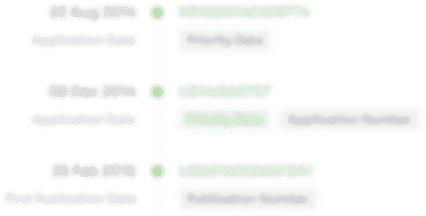
- R&D Engineer
- R&D Manager
- IP Professional
- Industry Leading Data Capabilities
- Powerful AI technology
- Patent DNA Extraction
Browse by: Latest US Patents, China's latest patents, Technical Efficacy Thesaurus, Application Domain, Technology Topic, Popular Technical Reports.
© 2024 PatSnap. All rights reserved.Legal|Privacy policy|Modern Slavery Act Transparency Statement|Sitemap|About US| Contact US: help@patsnap.com