Multi-park energy scheduling method and system based on deep reinforcement learning
A technology of reinforcement learning and energy scheduling, applied in neural learning methods, information technology support systems, resources, etc., can solve problems such as the difficulty of implementing an integrated energy system, promote photovoltaic consumption, improve effectiveness, and avoid sources that cannot respond in real time. The effect of random variation of the load
- Summary
- Abstract
- Description
- Claims
- Application Information
AI Technical Summary
Problems solved by technology
Method used
Image
Examples
Embodiment Construction
[0107] The present invention will be further described below in conjunction with the accompanying drawings.
[0108] refer to Figure 1 to Figure 9 , a multi-park energy scheduling method based on deep reinforcement learning, including the following steps:
[0109] S1: Build a distributed park integrated energy system model, propose an optimal dispatching framework for the distributed park integrated energy system with the goal of optimal economic operation, and design the energy interaction between each park, which is divided into multi-park sharing layer and single park consumption layer;
[0110] S2: On the basis of the proposed architecture, use a multi-agent-based deep reinforcement learning algorithm to solve the dynamic scheduling problem of the integrated energy system, and build a multi-agent deep reinforcement learning framework for the distributed park integrated energy system;
[0111] S3: Replacing the objective function with a real-time reward function, using t...
PUM
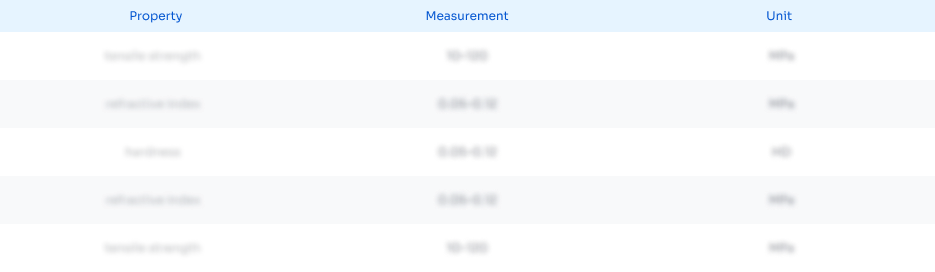
Abstract
Description
Claims
Application Information
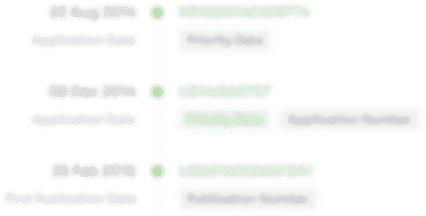
- R&D Engineer
- R&D Manager
- IP Professional
- Industry Leading Data Capabilities
- Powerful AI technology
- Patent DNA Extraction
Browse by: Latest US Patents, China's latest patents, Technical Efficacy Thesaurus, Application Domain, Technology Topic, Popular Technical Reports.
© 2024 PatSnap. All rights reserved.Legal|Privacy policy|Modern Slavery Act Transparency Statement|Sitemap|About US| Contact US: help@patsnap.com