Power system dynamic frequency response curve prediction method and system based on XGBoost
A frequency response curve and dynamic frequency technology, which is applied in the field of dynamic frequency response curve prediction based on XGBoost power system, can solve the problems of strong dependence on deep learning samples and slow offline training speed, and achieve the goal of reducing dependence and increasing output diversity. Effect
- Summary
- Abstract
- Description
- Claims
- Application Information
AI Technical Summary
Problems solved by technology
Method used
Image
Examples
Embodiment 1
[0044] Example 1, such as figure 1 As shown, a method for predicting the dynamic frequency response curve of a power system based on XGBoost, the steps are as follows:
[0045]S1. Obtain a diverse database for offline training based on the simulation results of massive predicted fault scenarios; among them, massive predicted fault scenarios include new energy off-grid faults, large-capacity unit trip faults, and DC blocking faults. The data in the diverse database includes input features and corresponding output dynamic frequency response values, where the input features are unit inertia time constant, unit start-stop status, active power disturbance, fault location, unit regulated power and spinning reserve Level.
[0046] The corresponding output dynamic frequency response value is to use the inertia center frequency to represent the system frequency dynamic response in the global state, and the specific formula is:
[0047]
[0048] where f COI Indicates the center fr...
Embodiment 2
[0099] Embodiment 2, a dynamic frequency response curve prediction system based on XGBoost power system, including a database construction module, a data normalization module, a frequency response curve predictor establishment module, a parameter adjustment module based on Bayesian optimization, and frequency response curve prediction The offline training module of the device and the online prediction module of the frequency response curve predictor, the database construction module is connected with the data normalization module, and the data normalization module is respectively connected with the parameter adjustment module based on Bayesian optimization and the frequency response curve predictor The offline training module is connected with the frequency response curve predictor online prediction module, the frequency response curve predictor establishment module is connected with the parameter adjustment module based on Bayesian optimization, and the frequency response curve...
PUM
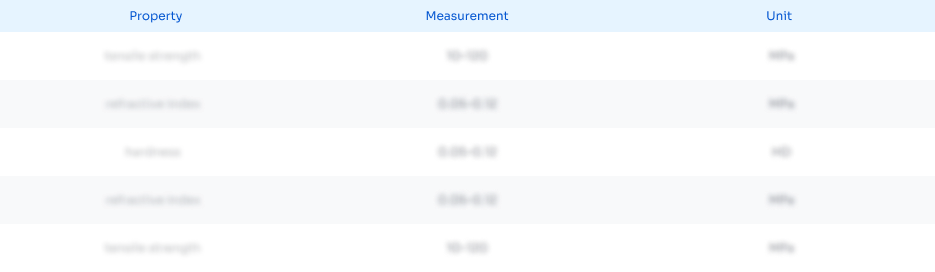
Abstract
Description
Claims
Application Information
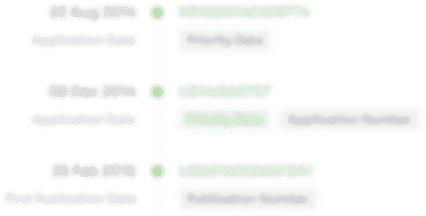
- R&D
- Intellectual Property
- Life Sciences
- Materials
- Tech Scout
- Unparalleled Data Quality
- Higher Quality Content
- 60% Fewer Hallucinations
Browse by: Latest US Patents, China's latest patents, Technical Efficacy Thesaurus, Application Domain, Technology Topic, Popular Technical Reports.
© 2025 PatSnap. All rights reserved.Legal|Privacy policy|Modern Slavery Act Transparency Statement|Sitemap|About US| Contact US: help@patsnap.com