Deep learning-based CT image endangered organ segmentation system
A CT image and deep learning technology, applied in the field of medical image processing, can solve problems such as reducing the level of network nonlinearity, pixel misclassification, and poor segmentation effect, so as to improve segmentation accuracy, strengthen learning ability, and enhance nonlinearity. Effect
- Summary
- Abstract
- Description
- Claims
- Application Information
AI Technical Summary
Problems solved by technology
Method used
Image
Examples
Embodiment Construction
[0050] The present invention will be further described in detail below in conjunction with the embodiments and the accompanying drawings, but the embodiments of the present invention are not limited thereto.
[0051] Such as figure 1 As shown, the deep learning-based CT image organ-at-risk segmentation system provided in this embodiment includes: a data acquisition module, a region-of-interest delineation module, an organ-at-risk segmentation model training module, a model testing module, and a segmented image generation module.
[0052] The data collection module is used to collect a CT image data set, and the CT image data set includes CT images containing multiple organs at risk of nasopharyngeal carcinoma. In this embodiment, the target part of the sample selection is concentrated on the head and neck due to pathological reasons; the CT image data set is randomly divided to form a training set and a test set, with a ratio of 4:1.
[0053] The region of interest delineatio...
PUM
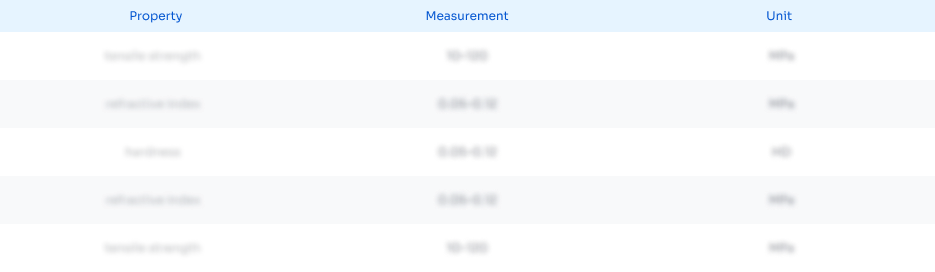
Abstract
Description
Claims
Application Information
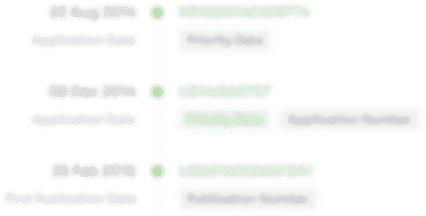
- R&D Engineer
- R&D Manager
- IP Professional
- Industry Leading Data Capabilities
- Powerful AI technology
- Patent DNA Extraction
Browse by: Latest US Patents, China's latest patents, Technical Efficacy Thesaurus, Application Domain, Technology Topic, Popular Technical Reports.
© 2024 PatSnap. All rights reserved.Legal|Privacy policy|Modern Slavery Act Transparency Statement|Sitemap|About US| Contact US: help@patsnap.com