Hybrid scheduling method based on maximum consumption of new energy and optimal power generation cost
A technology of power generation cost and hybrid scheduling, applied in the fields of genetic laws, genetic models, electrical components, etc., can solve the problems of insufficient utilization of clean energy, abandonment of wind and solar energy, etc., to achieve improved distribution range and uniformity, and accurate power generation costs. Effect
- Summary
- Abstract
- Description
- Claims
- Application Information
AI Technical Summary
Problems solved by technology
Method used
Image
Examples
Embodiment 1
[0113] Such as figure 1 As shown, a hybrid scheduling method based on the maximum consumption of new energy and the optimal cost of power generation, the steps include:
[0114] Step 1. Establish an optimization model based on new energy consumption capacity and power generation cost, construct the maximum objective function of new energy consumption and the minimum operating cost of the integrated energy system, energy balance constraints, system energy supply equipment constraints, and energy storage devices Constraints and spinning reserve constraints;
[0115] Step 2, set the population size pop, the number of iterations gen, the number of objective functions M, the number of decision variables V, the inertia weight w, the individual learning coefficient c1, the global learning coefficient c2, and the velocity vector fori;
[0116] Step 3, through the genetic algorithm of non-dominated sorting, iteratively solve the optimal value of the new energy consumption capacity and...
Embodiment 2
[0123] Such as figure 2 As shown, the operation steps of the non-dominated sorting genetic algorithm NSGA-II are as follows:
[0124] Step a: Firstly, random simulation operation is performed to generate an initial population P with a scale value of N, followed by the generation of offspring population Q, and then the combination of population P and offspring population Q, and finally a population M with a scale value of 2N;
[0125] Step b, combine the parent and child populations, perform non-dominated sorting, and calculate the crowding degree of all individuals in the non-dominated layer, and then select the appropriate individual from the non-dominated relationship and individual crowding degree to form a new parent Population P 1 ;
[0126] Step c, generate a new offspring population Q through a genetic algorithm, and transfer the new parent population P 1 with the new offspring population Q 1 combine to form a new population M 1 , repeat the above steps until the ...
Embodiment 3
[0128] The operation steps of the multi-objective particle swarm optimization algorithm MOPSO are as follows:
[0129] Step a, for each particle in the particle swarm, initialize its velocity and position;
[0130] Step b, calculate the target vector value of each particle, and add the non-dominated solution to the external memory;
[0131] Step c, determine the initial global best position gbest and individual best position pbest of the particle;
[0132] Step d, update the velocity and position of the particle, and take certain measures to ensure that the particle flies in the search space;
[0133] Step e, calculate the target vector of each particle, and adjust the individual best position pbest of the particle;
[0134] Step f, update the external memory, and select the global best position gbest for each particle at the same time;
[0135] Step g, judge whether the algorithm termination condition is satisfied, if satisfied, the algorithm stops running; otherwise, retu...
PUM
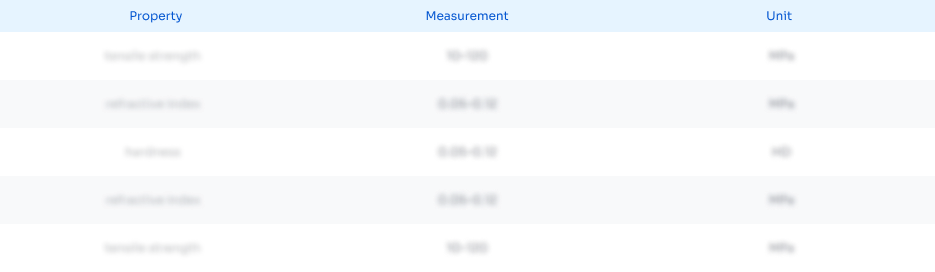
Abstract
Description
Claims
Application Information
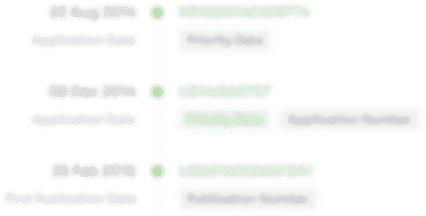
- R&D Engineer
- R&D Manager
- IP Professional
- Industry Leading Data Capabilities
- Powerful AI technology
- Patent DNA Extraction
Browse by: Latest US Patents, China's latest patents, Technical Efficacy Thesaurus, Application Domain, Technology Topic, Popular Technical Reports.
© 2024 PatSnap. All rights reserved.Legal|Privacy policy|Modern Slavery Act Transparency Statement|Sitemap|About US| Contact US: help@patsnap.com