Traffic flow prediction parallel method based on chaos and reinforcement learning
A technology of reinforcement learning and traffic flow, applied in the field of traffic flow prediction based on chaos and reinforcement learning, can solve problems such as insufficient parallelization and complex structure, achieve fast learning and adjustment, best prediction results, strong interpretability and the effect of the ability to adjust online
- Summary
- Abstract
- Description
- Claims
- Application Information
AI Technical Summary
Problems solved by technology
Method used
Image
Examples
example 1
[0099] Example 1: Preprocessing of traffic flow data.
[0100] Step1_1, set the embedding dimension m=3 and time delay k=2 of the initialized chaotic time series;
[0101] Step1_2, calculate the maximum Lyapunov exponent of the reconstructed traffic flow time series data by Wolf method, and analyze the chaotic characteristics of the traffic flow time series;
[0102] Step1_3, initialize the chaotic model and generate a comparative chaotic time series, the chaotic model is X i+1 =4X i (1-X i ), where X 1 = 0.1;
[0103] Step1_4, initialize reconstruction and compare the embedding dimension md=3 and time delay kd=2 of chaotic time series data;
[0104] Step1_5, standardize the traffic flow time series and comparative chaotic time series, set the traffic flow time series data and comparative chaotic time series as follows:
[0105] T = [3,6,8,5,7,10,5];
[0106] Td = [0.3600, 0.9216, 0.2890, 0.8219, 0.5854, 0.9708, 0.1133];
[0107]Among them, the mean value of traffic fl...
example 2
[0118] Example 2: Constructing a reinforcement learning environment.
[0119] Step2_1, the data of the preprocessed traffic flow data training set is used as the state space in the environment and sequenced according to time
[0120] Arranged in order, the settings are as follows:
[0121] S 1 =T1=[-0.67,0.34,0.14],
[0122] S 2 =T2=[-0.06,-0.27,0.74],
[0123] S 3 =T3=[0.34,0.14,-0.27],
[0124] …,
[0125] S n =Tn=[0.64,0.25,-0.56];
[0126] Step2_2, perform difference operation on the last one-dimensional data of the adjacent state space in turn to obtain the range of the action space, that is, set:
[0127] T=[3,6,8,5,7,10,5,…,9,10],
[0128] t c1 =10-7=3,
[0129] t c2 =5-10=-5,
[0130] …,
[0131] t cn =10-9=1,
[0132] Then set the action space range as: [-5,3], and the standard deviation of the difference is 0.36;
[0133] Step2_3, with t ci As the center, the reward of the action space corresponding to the state Si is distributed according to the n...
example 3
[0153] Example 3: Initialize the neural network model structure and update method.
[0154] Step3_1, initialize the actor network structure. Since the actor network is used to estimate the action strategy of the agent and the strategy is continuous, the number of input neurons of the actor network is m, which is the state dimension of the environment. When the environment is a training environment, m is the training environment. The state dimension of the environment. When the environment is a comparison environment, m is the state dimension of the comparison environment. The middle layer network structure uses a neural network with a CRU structure, and the output is [d min , d max ] and use the softmax activation function to build the model, for example, if the reconstructed state is set to S=[-0.59,0.42,0.38,0.81], and the action space is [-2,3], then the input neuron The number is 4, the optional action is [-2, -1, 0, 1, 2, 3], and the probability distribution of the corre...
PUM
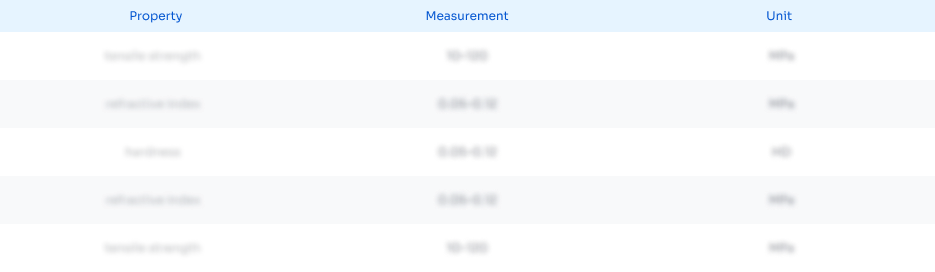
Abstract
Description
Claims
Application Information
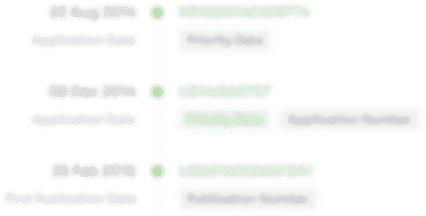
- Generate Ideas
- Intellectual Property
- Life Sciences
- Materials
- Tech Scout
- Unparalleled Data Quality
- Higher Quality Content
- 60% Fewer Hallucinations
Browse by: Latest US Patents, China's latest patents, Technical Efficacy Thesaurus, Application Domain, Technology Topic, Popular Technical Reports.
© 2025 PatSnap. All rights reserved.Legal|Privacy policy|Modern Slavery Act Transparency Statement|Sitemap|About US| Contact US: help@patsnap.com