End-to-end brain causal network construction method based on graph neural network
A causal network and neural network technology, applied in the field of end-to-end brain causal network construction, can solve problems such as the inability of the model to adapt to the brain signal noise environment, model generalization, and computational cost limitations, so as to avoid insufficient information mining and better The effect of generalization and high accuracy
- Summary
- Abstract
- Description
- Claims
- Application Information
AI Technical Summary
Problems solved by technology
Method used
Image
Examples
Embodiment Construction
[0043] The implementation examples of the present invention will be further described below in conjunction with the accompanying drawings.
[0044] see figure 1 , the present invention proposes an end-to-end brain causal network estimation method based on graph neural network, which is realized by the following steps:
[0045] Step S1: Use the vector autoregressive model to construct a time-series causal simulated EEG signal with EEG signal characteristics as the input of the model, and obtain a predefined causal network as a label for calculating the loss function;
[0046] Step S2: Design a graph neural network model of a multi-layer perceptron based on feature fusion of adjacent k layers. For the designed graph neural network model, please refer to figure 2 , the multi-layer perceptron structure for feature fusion of adjacent k layers, please refer to image 3 ;
[0047] Step S3: Add noise with different signal-to-noise ratios to the simulated EEG signal obtained in st...
PUM
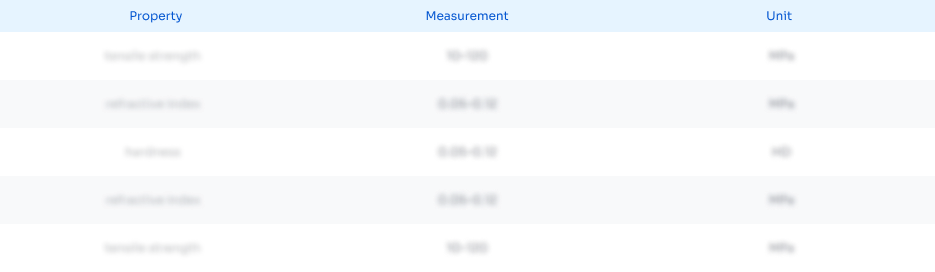
Abstract
Description
Claims
Application Information
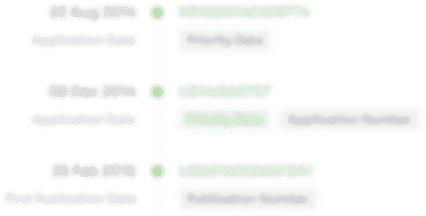
- R&D Engineer
- R&D Manager
- IP Professional
- Industry Leading Data Capabilities
- Powerful AI technology
- Patent DNA Extraction
Browse by: Latest US Patents, China's latest patents, Technical Efficacy Thesaurus, Application Domain, Technology Topic, Popular Technical Reports.
© 2024 PatSnap. All rights reserved.Legal|Privacy policy|Modern Slavery Act Transparency Statement|Sitemap|About US| Contact US: help@patsnap.com