Building wind field ground roughness identification method based on multi-scale input neural network
A technology of ground roughness and neural network is applied in the field of ground roughness recognition of building wind farms based on multi-scale input neural network, which can solve the problems of low recognition accuracy and poor execution efficiency, achieve accurate classification results and improve robustness. , the effect of high classification efficiency
- Summary
- Abstract
- Description
- Claims
- Application Information
AI Technical Summary
Problems solved by technology
Method used
Image
Examples
Embodiment Construction
[0028] The technical solution of the present invention will be described in detail below in conjunction with the drawings and embodiments, so that those skilled in the art can better understand and implement the technical solution of the present invention.
[0029] The ground roughness recognition method of building wind field based on multi-scale input neural network, such as Figure 1 to Figure 3 As shown, it mainly includes building data sets, classification model training and model testing, specifically including the following steps:
[0030] S1. Divide three scales according to the size of the surrounding area of the building. In this embodiment, the sample image is divided into three scales of 10Km, 5Km, and 2Km. Satellite images of different scales around the building are collected to obtain sample images, and the sample images are preprocessed It is a circle, and the circular sample image is divided into 36 fan-shaped images. The satellite image can be directly inter...
PUM
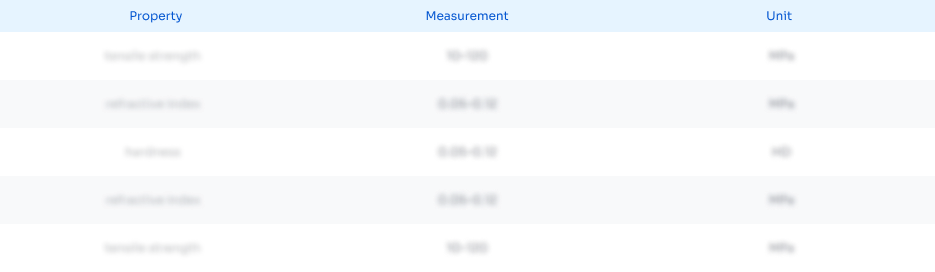
Abstract
Description
Claims
Application Information
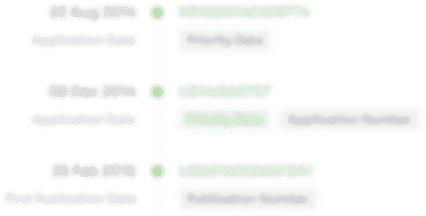
- R&D Engineer
- R&D Manager
- IP Professional
- Industry Leading Data Capabilities
- Powerful AI technology
- Patent DNA Extraction
Browse by: Latest US Patents, China's latest patents, Technical Efficacy Thesaurus, Application Domain, Technology Topic, Popular Technical Reports.
© 2024 PatSnap. All rights reserved.Legal|Privacy policy|Modern Slavery Act Transparency Statement|Sitemap|About US| Contact US: help@patsnap.com