Emotion prediction method and system based on interactive double-graph convolutional network
A technology of convolutional network and prediction method, applied in the field of emotion prediction method and system based on interactive double graph convolutional network, can solve the problems of informal expression online comments, syntactic structure errors, complexity, etc., to improve accuracy and stability, the effect of solving syntax errors
- Summary
- Abstract
- Description
- Claims
- Application Information
AI Technical Summary
Problems solved by technology
Method used
Image
Examples
Embodiment 1
[0045] This embodiment provides an emotion prediction method based on an interactive dual graph convolutional network, such as figure 1 shown, including the following steps:
[0046] Step 1: Obtain the text to be predicted, perform word embedding training processing on the text to be predicted, and convert the text into word vector embedding, that is, to obtain the word vector sequence of each word in the text.
[0047] Specifically, the GloVe word embedding tool is used. given a context sequence consisting of m words and an aspect sequence consisting of n words Among them, W a is W c subsequence. Via pretrained GloVe embedding matrix where d m represents the embedding dimension of the word vector, and |V| represents the size of the vocabulary.
[0048] Step 2: Convert several word vector sequences obtained in step 1 into contextual representations, that is, the hidden state vector H c ={h 1 ,h 2 ,...,h n }. Specifically, several word vector sequences are input...
Embodiment 2
[0088] This embodiment provides an emotion prediction system based on an interactive dual graph convolutional network, which specifically includes the following modules:
[0089] A text conversion module, which is configured to: obtain the text to be predicted and convert it into several word vector sequences;
[0090] a context information extraction module, which is configured to: extract the context information of several word vector sequences to obtain a hidden state vector;
[0091] A syntactic graph convolution module, which is configured to: obtain a syntactic graph by using a syntactic graph convolution network based on several word vector sequences;
[0092] a semantic graph convolution module, which is configured to: obtain a semantic graph by using a semantic graph convolution network based on the hidden state vector;
[0093] The interactive learning module is configured to: based on the syntactic graph and the semantic graph, interactively learn the syntactic inf...
Embodiment 3
[0097] This embodiment provides a computer-readable storage medium on which a computer program is stored, and when the program is executed by a processor, implements the emotion prediction method based on the interactive dual graph convolutional network as described in the first embodiment above A step of.
PUM
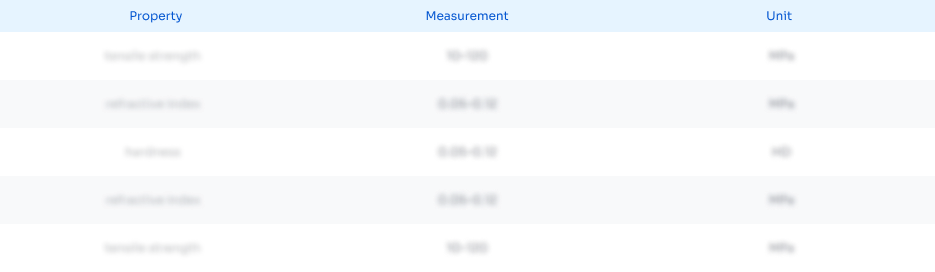
Abstract
Description
Claims
Application Information
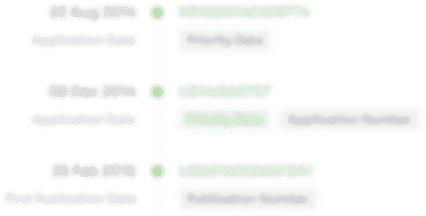
- R&D
- Intellectual Property
- Life Sciences
- Materials
- Tech Scout
- Unparalleled Data Quality
- Higher Quality Content
- 60% Fewer Hallucinations
Browse by: Latest US Patents, China's latest patents, Technical Efficacy Thesaurus, Application Domain, Technology Topic, Popular Technical Reports.
© 2025 PatSnap. All rights reserved.Legal|Privacy policy|Modern Slavery Act Transparency Statement|Sitemap|About US| Contact US: help@patsnap.com