Neural network accelerator fault vulnerability assessment method based on hardware feature information
A technology of hardware feature information and neural network, which is applied in the field of neural network accelerator fault vulnerability assessment based on hardware feature information, can solve the problems of less verification work and low accuracy of network layer-by-layer robustness, and achieve security, Refine for fine-grained effects
- Summary
- Abstract
- Description
- Claims
- Application Information
AI Technical Summary
Problems solved by technology
Method used
Image
Examples
Embodiment
[0084] In order to verify the effectiveness of a method for evaluating the fault vulnerability of a neural network accelerator based on hardware feature information of the present invention, experiments were carried out. refer to image 3 , the target neural network is selected as VGG16, and the fault category is clock glitch fault.
[0085] image 3 a is the effect of using Johnson's SU formula to fit the damage probability of data blocks. It can be seen from the figure that Johnson's SU can perfectly fit the damage probability distribution of actual hardware data blocks. image 3 b is the difference between the performance of software simulated faults and the performance of hardware faults in actual scenarios. It can be seen that the differences between most of the layers in VGG16 are within 0.1, indicating the accuracy of software simulated faults. image 3 c is the result of inter-layer search of VGG16. It can be seen that VGG16 has high vulnerability at layers 1, 14, an...
PUM
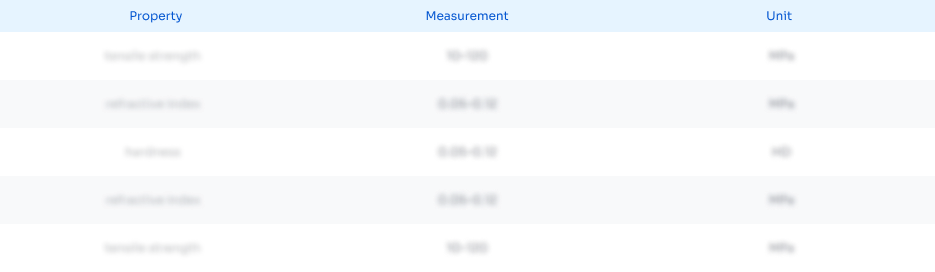
Abstract
Description
Claims
Application Information
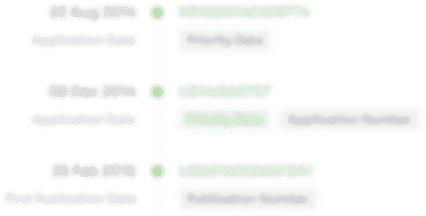
- R&D Engineer
- R&D Manager
- IP Professional
- Industry Leading Data Capabilities
- Powerful AI technology
- Patent DNA Extraction
Browse by: Latest US Patents, China's latest patents, Technical Efficacy Thesaurus, Application Domain, Technology Topic, Popular Technical Reports.
© 2024 PatSnap. All rights reserved.Legal|Privacy policy|Modern Slavery Act Transparency Statement|Sitemap|About US| Contact US: help@patsnap.com