Rapid relation extraction method based on convolutional neural network and improved cascade labeling
A technology of convolutional neural network and relational extraction, which is applied in the field of fast relational extraction based on convolutional neural network and improved cascade labeling, can solve the problems of slow model training and prediction, and achieve good generalization, stability, and good The effect of speed and performance, good application prospect and promotion range
- Summary
- Abstract
- Description
- Claims
- Application Information
AI Technical Summary
Problems solved by technology
Method used
Image
Examples
Embodiment 1
[0116] As a specific embodiment of the present invention, the present invention provides training and prediction of a fast relation extraction method based on convolutional neural network and improved cascade annotation as shown in the following table for the training configuration flow table.
[0117]
[0118] The fast relation extraction model is used to train and predict relation extraction tasks on real Chinese and English data sets, and all other embodiments use the same data as this embodiment. Among them, the Chinese data set uses the DuIE relation extraction training set released by Baidu, which contains 11958 training data, 1498 verification data and 1489 test data, and defines 48 different relationship types; the English data set uses the New York Times corpus and The NYT relation extraction dataset obtained by Freebase's remote supervision contains 70,339 training data and 4,006 test data, and defines 29 different relation types. Due to the good robustness and ge...
Embodiment 2
[0121] The fast relation extraction model based on convolutional neural network and improved cascade annotation has fast reasoning ability. First of all, the model has less parameters, which is about 1% of the parameters of other relation extraction models at present, so the model requires calculation The time and computing resources are greatly reduced; when extracting relational triples in real-world natural text, the model takes less than 5ms on average to process a natural text, and the speed is 7-15 times faster than other methods.
[0122] In practical applications, it is often required that the model can process many natural texts at a time to ensure the parallelism of model operations. In the case of limited computing resources, the traditional relation extraction method using pre-trained language model as an encoder can only process and extract less than 8 or even 4 natural texts at a time, while the fast relation extraction model is limited by the amount of parameters...
Embodiment 3
[0124] The fast relation extraction model based on convolutional neural network and improved cascade annotation is easy to train and takes less time to train. Compared with other relation extraction methods, it has shorter turnaround time for single parameter update and less total parameters Update turnaround times so less total training time is required. For example, when the amount of training data is about 70,000, the batch size is set to 32, and the model is trained for 60 epochs, and it only takes about 100 minutes in the end, which is 3 to 10 times faster than other methods.
PUM
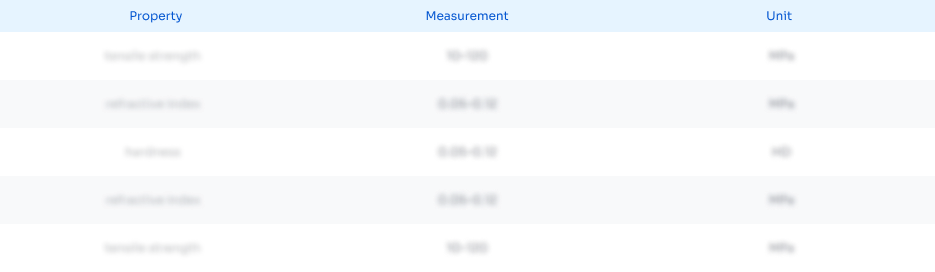
Abstract
Description
Claims
Application Information
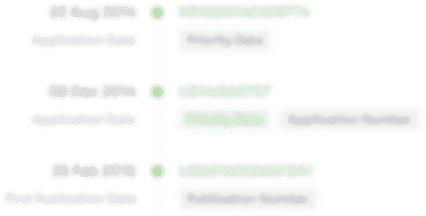
- R&D Engineer
- R&D Manager
- IP Professional
- Industry Leading Data Capabilities
- Powerful AI technology
- Patent DNA Extraction
Browse by: Latest US Patents, China's latest patents, Technical Efficacy Thesaurus, Application Domain, Technology Topic, Popular Technical Reports.
© 2024 PatSnap. All rights reserved.Legal|Privacy policy|Modern Slavery Act Transparency Statement|Sitemap|About US| Contact US: help@patsnap.com