Low-illumination image enhancement model and method, electronic equipment and storage medium
An image enhancement, low-light technology, applied in the field of image processing, can solve the problem of unsatisfactory image enhancement effect, and achieve the effect of improving robustness, ensuring interpretability, and ensuring flexibility
- Summary
- Abstract
- Description
- Claims
- Application Information
AI Technical Summary
Problems solved by technology
Method used
Image
Examples
Embodiment 1
[0046] Figure 1A The structure of the low-light image enhancement model provided by the first embodiment of the present invention is shown. The low-light image enhancement model includes a low-light image enhancement model including an initialization module 11, an optimization module 12, an illumination adjustment module 13, and an image reconstruction module connected in sequence. 14; Wherein, the initialization module is used to initialize and decompose the input image, and obtain the initialization illumination layer and the initialization reflection layer corresponding to the input image; , to obtain the optimized illumination layer and the optimized reflection layer; the illumination adjustment module is used to adjust the illumination of the optimized illumination layer to obtain the target illumination layer; the image reconstruction module is used to reconstruct the image according to the target illumination layer and the optimized reflection layer to obtain the target ...
Embodiment 2
[0100] This embodiment further illustrates the low-light enhancement model described in Experimental Example 1 in combination with the experimental example:
[0101] This experimental example subjectively and objectively evaluates the unfolding-based low-light image enhancement model described in Embodiment 1 on two public low-light image enhancement test sets. The two representative datasets are the LOL dataset and the SICE dataset, respectively. This experimental example uses common reference indicators to evaluate image quality, namely MeanAbsolute Error (MAE), Structural Similarity (SSIM), Peak Signal to Noise Ratio (PSNR) and Learned Perceptual Image Patch Similarity (LPIPS). A good model should have high PSNR and SSIM metric scores, but low MAE and LPIPS scores. This experimental example compares the low-light image enhancement model proposed in Example 1 with some existing benchmark models, these benchmark models are LIME, NPE, SRIE, RRM, LR3M, Retinex-Net, KinD, Zero-...
Embodiment 3
[0112] Embodiment 3 of the present invention is implemented based on the low-light image enhancement model described in Embodiment 1, image 3 The implementation process of the low-light image enhancement method provided by the third embodiment of the present invention is shown. For the convenience of description, only the part related to the embodiment of the present invention is shown, and the details are as follows:
[0113] In step S301, the input image is initialized and decomposed by the initialization module, and the initialized illumination layer and the initialized reflection layer corresponding to the input image are obtained.
[0114] In the embodiment of the present invention, the above-mentioned input image is a low-light image to be image-enhanced. Considering that variable initialization plays an important role for iterative optimization algorithms (such as ADMM), the input image can be initialized and decomposed using commonly used all-zero initialization decom...
PUM
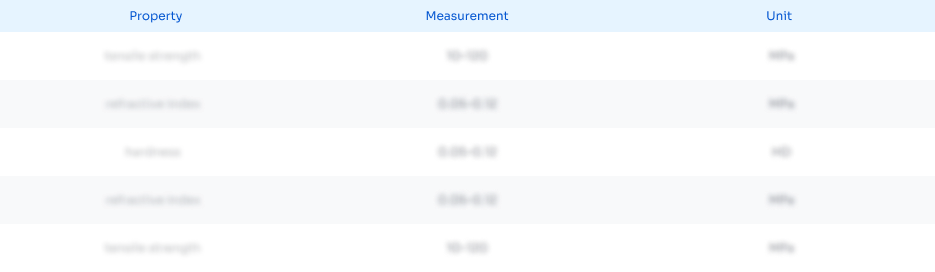
Abstract
Description
Claims
Application Information
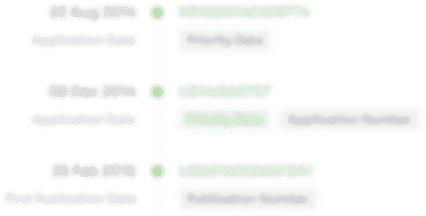
- R&D Engineer
- R&D Manager
- IP Professional
- Industry Leading Data Capabilities
- Powerful AI technology
- Patent DNA Extraction
Browse by: Latest US Patents, China's latest patents, Technical Efficacy Thesaurus, Application Domain, Technology Topic, Popular Technical Reports.
© 2024 PatSnap. All rights reserved.Legal|Privacy policy|Modern Slavery Act Transparency Statement|Sitemap|About US| Contact US: help@patsnap.com