Short-term photovoltaic power prediction method based on self-encoder deep learning model
A technology of deep learning and autoencoder, which is applied in instrumentation, design optimization/simulation, calculation, etc., to achieve better prediction performance and better prediction performance
- Summary
- Abstract
- Description
- Claims
- Application Information
AI Technical Summary
Problems solved by technology
Method used
Image
Examples
Embodiment 1
[0061] Provides short-term photovoltaic power prediction methods based on autoencoder deep learning models, including:
[0062] S1, build a variational autoencoder model VAE;
[0063] S2, short-term photovoltaic power prediction is performed based on the variational autoencoder model VAE.
[0064] As a preferred embodiment, the construction of the variational autoencoder VAE is based on deep learning technology, which has a good ability in automatically learning relevant features of embedded data and can be used to predict photovoltaic power output. Variational Autoencoders Model (VAE) is a class of generative-based techniques that can efficiently and automatically extract information from data in an unsupervised manner. A desirable property of VAEs is their ability to reduce input dimensionality and are very effective for approximating complex data distributions using stochastic gradient descent. Compared with traditional autoencoders, VAE uses an adjustment mechanism durin...
specific Embodiment
[0096] 1. Photovoltaic power station data
[0097] In this embodiment, data from a solar photovoltaic power station in Guizhou is used to verify the performance of the deep learning model prediction method. The real-time monitoring irradiance and actual power curve of photovoltaic power station are as follows: Figure 5 (a) and Figure 5 (b). The time resolution of the photovoltaic power plant is 15 minutes. The power station will be fully put into operation in July 2021, with an installed capacity of 50MW, and the data collected will be from August 1, 2021 to October 16, 2020.
[0098] Image 6 (a)-(d) depict the irradiance, ambient temperature, component surface temperature and corresponding DC output of the power station on a certain day. Observe how the average irradiance, ambient temperature, module temperature and DC power first increase and then decrease from sunrise to sunset. Generally, module temperature increases with temperature and irradiation.
[0099] 2. ...
Embodiment 2
[0120] A short-term photovoltaic power prediction system based on an autoencoder deep learning model, including:
[0121] a model building block for building a variational autoencoder model VAE; and
[0122] A power prediction module, configured to perform short-term photovoltaic power prediction based on the variational autoencoder model VAE.
[0123] The present invention also provides a memory that stores a plurality of instructions, and the instructions are used to implement the method described in the first embodiment.
[0124] like Figure 10 As shown, the present invention also provides an electronic device, comprising a processor 301 and a memory 302 connected to the processor 301, the memory 302 stores a plurality of instructions, the instructions can be loaded by the processor and is executed, so that the processor can execute the method described in the first embodiment.
PUM
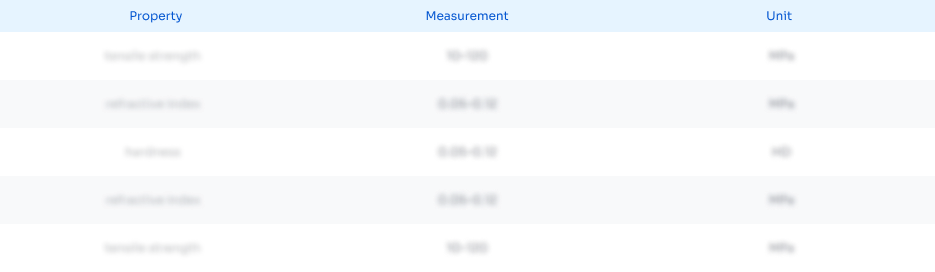
Abstract
Description
Claims
Application Information
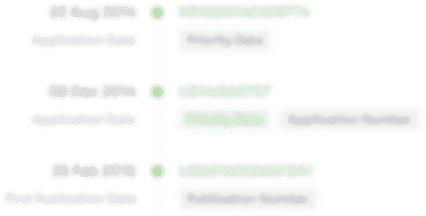
- R&D Engineer
- R&D Manager
- IP Professional
- Industry Leading Data Capabilities
- Powerful AI technology
- Patent DNA Extraction
Browse by: Latest US Patents, China's latest patents, Technical Efficacy Thesaurus, Application Domain, Technology Topic, Popular Technical Reports.
© 2024 PatSnap. All rights reserved.Legal|Privacy policy|Modern Slavery Act Transparency Statement|Sitemap|About US| Contact US: help@patsnap.com