Engine residual life prediction method of multi-period rule cascade memory network
A life prediction and engine technology, applied in the prediction of the remaining life of the engine, multi-period rule cascaded memory network prediction field, can solve the problem of reducing the volatility of the prediction results of the remaining life of the engine, the inability to accurately predict the remaining life of the engine, and the inability to accurately fit the engine Remaining life and other issues to achieve the effect of improving prediction effect, good prediction efficiency, and improving prediction accuracy
- Summary
- Abstract
- Description
- Claims
- Application Information
AI Technical Summary
Problems solved by technology
Method used
Image
Examples
Embodiment Construction
[0049] The present invention proposes a method for predicting the remaining life of an engine of a multi-period regular cascade memory network, which is described in detail as follows with reference to the accompanying drawings:
[0050] figure 1 It is the frame diagram of the automatic expansion and cascaded long short-term memory network described in the method of the present invention. Depend on figure 1 As shown in the figure, the automatically extended cascaded long-term and short-term memory network is composed of multiple sub-modules with the same structure connected step by step, and each sub-module includes a long-term and short-term memory layer, multiple fully connected layers and a regression output layer. The training set data is input in time series, and normalized to the range of 0-1, and then after the training of the long and short-term memory layer, the fully connected layer, and the regression output layer, the trained sub-module is obtained. Then, using t...
PUM
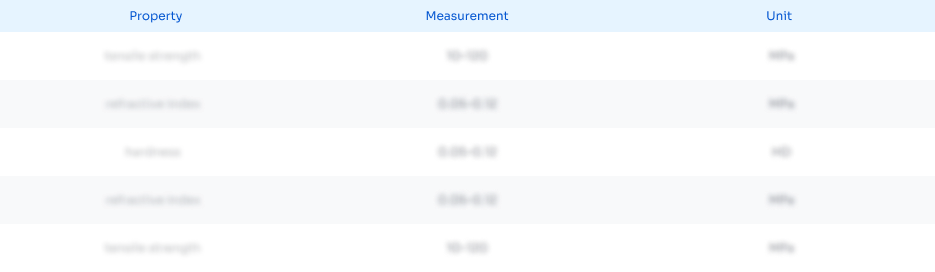
Abstract
Description
Claims
Application Information
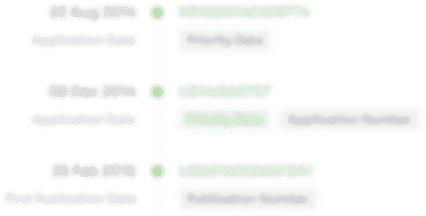
- R&D Engineer
- R&D Manager
- IP Professional
- Industry Leading Data Capabilities
- Powerful AI technology
- Patent DNA Extraction
Browse by: Latest US Patents, China's latest patents, Technical Efficacy Thesaurus, Application Domain, Technology Topic, Popular Technical Reports.
© 2024 PatSnap. All rights reserved.Legal|Privacy policy|Modern Slavery Act Transparency Statement|Sitemap|About US| Contact US: help@patsnap.com