Multi-modal feature fusion MRI brain tumor image segmentation method based on attention mechanism
A feature fusion and image segmentation technology, applied in the field of deep learning, can solve the problems of increasing information, increasing the necessary information for segmentation, and increasing the difficulty of segmentation problems, so as to improve the accuracy, expand the receptive field, and solve the effect of low boundary contrast.
- Summary
- Abstract
- Description
- Claims
- Application Information
AI Technical Summary
Problems solved by technology
Method used
Image
Examples
Embodiment Construction
[0048] The present invention will be further described below in conjunction with the accompanying drawings:
[0049] like figure 1 As shown, a new lung CT image segmentation method based on transfer learning and attention mechanism of the present invention specifically includes the following steps:
[0050] Step 1) Input dataset;
[0051] Input MRI brain tumor image dataset BraTS2021. The Brain Tumor Segmentation Challenge (BraTS) is an annual international competition held since 2012. Participants were provided with a large number of fully annotated, multi-institution, multimodal MRI images of patients with gliomas of varying degrees. The magnetic resonance image modalities in the BraTS2021 dataset include four modalities: T1-weighted imaging, T2-weighted imaging, T1ce imaging, and free water suppression sequence (FLAIR).
[0052] Input the 2D multimodal MRI brain tumor image to be segmented.
[0053] Step 2) data augmentation and data preprocessing;
[0054] By slicing...
PUM
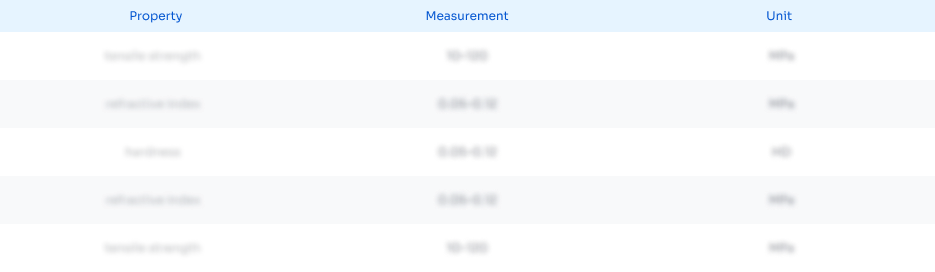
Abstract
Description
Claims
Application Information
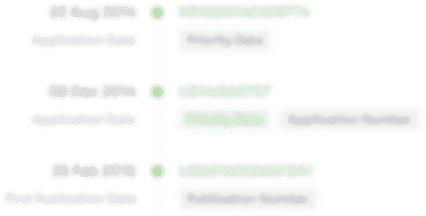
- R&D
- Intellectual Property
- Life Sciences
- Materials
- Tech Scout
- Unparalleled Data Quality
- Higher Quality Content
- 60% Fewer Hallucinations
Browse by: Latest US Patents, China's latest patents, Technical Efficacy Thesaurus, Application Domain, Technology Topic, Popular Technical Reports.
© 2025 PatSnap. All rights reserved.Legal|Privacy policy|Modern Slavery Act Transparency Statement|Sitemap|About US| Contact US: help@patsnap.com