Data-mechanism driven material attribute prediction method of graph neural network
A neural network and material property technology, which is applied in the fields of material discovery and graph neural network, can solve the problems of ignoring material properties in computational models and low generalization performance of deep learning networks, and achieve the effect of improving accuracy
- Summary
- Abstract
- Description
- Claims
- Application Information
AI Technical Summary
Problems solved by technology
Method used
Image
Examples
Embodiment 1
[0079] In this example, a well-collected molecular dataset is used to validate the effectiveness of the graph neural network's data-mechanism-driven material property prediction method. Molecular dataset includes 4208 pieces of data, each molecule includes: graph structure, descriptor, experimental value and calculated value. The graph structure includes the characteristics of the atoms and the characteristics of the bonds in the graph. Descriptors include: relative molecular mass, relative mass of heavy atoms, number of amino and hydroxyl groups, number of nitro groups, number of hydrogen acceptors, number of hydrogen donors, number of rotatable bonds, number of valence electrons, relative molecular mass, relative number of heavy atoms Mass, number of amino and hydroxyl groups. Molecular datasets are collected using methods such as image 3 shown.
[0080] The batch size of this model training is 32, the parameters are optimized using the Adam optimizer and the initial lea...
PUM
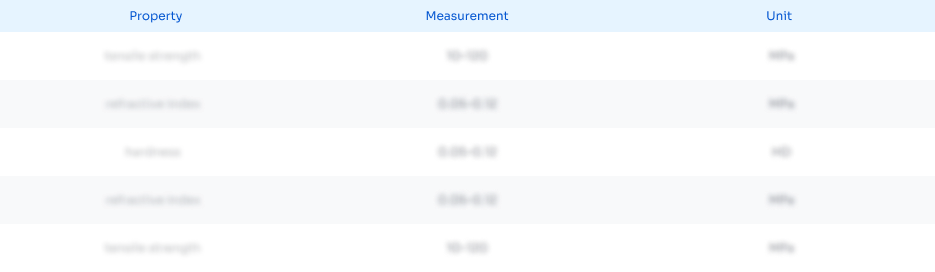
Abstract
Description
Claims
Application Information
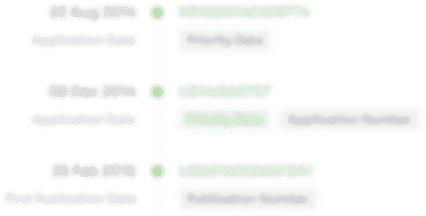
- R&D Engineer
- R&D Manager
- IP Professional
- Industry Leading Data Capabilities
- Powerful AI technology
- Patent DNA Extraction
Browse by: Latest US Patents, China's latest patents, Technical Efficacy Thesaurus, Application Domain, Technology Topic, Popular Technical Reports.
© 2024 PatSnap. All rights reserved.Legal|Privacy policy|Modern Slavery Act Transparency Statement|Sitemap|About US| Contact US: help@patsnap.com