Multi-modal breast cancer classification training method and system based on graph attention network
A training method and attention technology, which is applied in the field of disease classification and deep learning, can solve the problems that modal complementarity is not fully utilized, breast cancer is difficult to meet the requirements of clinical diagnosis, and achieve the effect of improving classification performance
- Summary
- Abstract
- Description
- Claims
- Application Information
AI Technical Summary
Problems solved by technology
Method used
Image
Examples
Embodiment Construction
[0061] In order to make the objectives, technical solutions and advantages of the present invention clearer, the present invention will be further described in detail below with reference to the accompanying drawings. Based on the embodiments of the present invention, all other embodiments obtained by persons of ordinary skill in the art without creative efforts shall fall within the protection scope of the present invention. It should be understood that the specific embodiments described herein are only used to explain the present invention, but not to limit the present invention.
[0062] combine figure 1 A schematic flowchart of the first embodiment of the present invention, the present invention proposes a breast cancer classification training method based on a graph attention network, which mainly includes the following steps:
[0063] Step 1, extracting representative pathological features from the patient's electronic medical record EMR, digitizing each feature, and pr...
PUM
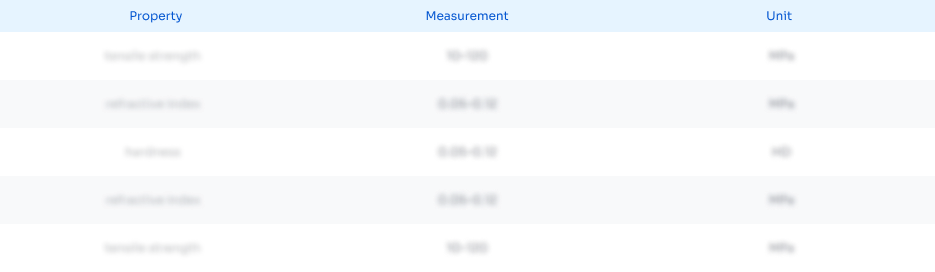
Abstract
Description
Claims
Application Information
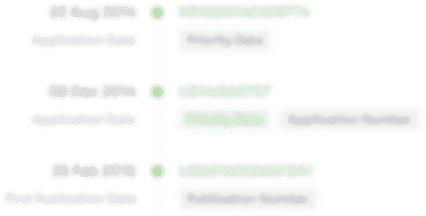
- R&D
- Intellectual Property
- Life Sciences
- Materials
- Tech Scout
- Unparalleled Data Quality
- Higher Quality Content
- 60% Fewer Hallucinations
Browse by: Latest US Patents, China's latest patents, Technical Efficacy Thesaurus, Application Domain, Technology Topic, Popular Technical Reports.
© 2025 PatSnap. All rights reserved.Legal|Privacy policy|Modern Slavery Act Transparency Statement|Sitemap|About US| Contact US: help@patsnap.com