Gas supply and demand dynamic prediction system for steel enterprises and method thereof
A dynamic forecasting and gas technology, applied in the direction of electrical program control, comprehensive factory control, comprehensive factory control, etc., can solve problems such as slow convergence speed, large error, and not allowed to speculate on independent variables, so as to overcome limitations and improve accuracy Effects on Sex and Reliability
- Summary
- Abstract
- Description
- Claims
- Application Information
AI Technical Summary
Problems solved by technology
Method used
Image
Examples
Embodiment Construction
[0028] Figure 1 ~ Figure 3 It is a specific embodiment of the present invention.
[0029] 1) Combined with the actual situation on site, the gas data collection is carried out.
[0030] 2) Perform the data processing required for modeling on the collected gas data.
[0031] 3) Carry out system modeling for the data that has been preprocessed. When modeling the system, you should first select the modeling method (different modeling methods are suitable for different situations), then select the analysis independent variables and dependent variables participating in the modeling, set the parameters required for modeling, and then click Modeling button to model the system.
[0032] 4) After modeling, you can directly observe the modeling effect directly on the modeling interface, and the modeling effect can be viewed through the modeling effect diagram. If the modeling effect is not ideal, it should be re-modeled.
[0033] 5) Carry out the model evaluation operation for the...
PUM
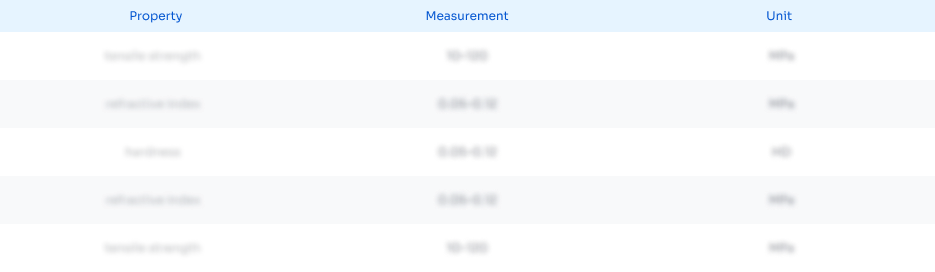
Abstract
Description
Claims
Application Information
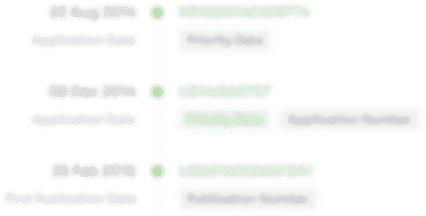
- R&D Engineer
- R&D Manager
- IP Professional
- Industry Leading Data Capabilities
- Powerful AI technology
- Patent DNA Extraction
Browse by: Latest US Patents, China's latest patents, Technical Efficacy Thesaurus, Application Domain, Technology Topic, Popular Technical Reports.
© 2024 PatSnap. All rights reserved.Legal|Privacy policy|Modern Slavery Act Transparency Statement|Sitemap|About US| Contact US: help@patsnap.com