Target tracking method based on Markov chain Monte-Carlo particle filtering
A Markov chain Monte Carlo and particle filter technology, applied in image data processing, instruments, image data processing, etc., can solve the problems of loss of particle diversity, particle degradation, sample impoverishment, etc., and achieve sample impoverishment problems, reducing poverty, improving diversity outcomes
- Summary
- Abstract
- Description
- Claims
- Application Information
AI Technical Summary
Problems solved by technology
Method used
Image
Examples
Embodiment 1
[0062] The univariate unsteady growth model (UNGM) is a nonlinear model widely used in the economic field. This model is used to compare the tracking performance of the PF-MCMC filter method with the general particle filter performance. One of the standard verification procedures for the algorithm performance of this particle filter, its state equation and observation equation are as follows:
[0063] x t = x k - 1 2 + 25 x k - 1 1 + x k - 1 2 + 8 cos ( 1.2 t ) ...
Embodiment 2
[0072] The following nonlinear model is used to verify the filter tracking performance, and its state equation and observation equation are as follows:
[0073] x k = 1 + sin ( ( 4 e - 2 ) π ( k - 1 ) ) + 0.5 x k - 1 + v k ...
PUM
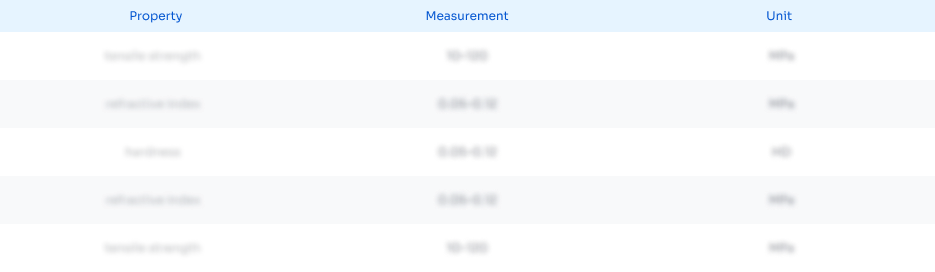
Abstract
Description
Claims
Application Information
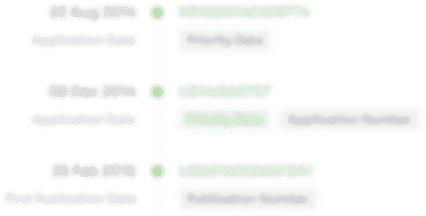
- R&D Engineer
- R&D Manager
- IP Professional
- Industry Leading Data Capabilities
- Powerful AI technology
- Patent DNA Extraction
Browse by: Latest US Patents, China's latest patents, Technical Efficacy Thesaurus, Application Domain, Technology Topic, Popular Technical Reports.
© 2024 PatSnap. All rights reserved.Legal|Privacy policy|Modern Slavery Act Transparency Statement|Sitemap|About US| Contact US: help@patsnap.com