Target retrieval method based on group of randomized visual vocabularies and context semantic information
A visual dictionary and semantic information technology, applied in computer components, secure communication devices, character and pattern recognition, etc., can solve problems such as high computational complexity, achieve enhanced differentiation, reduce semantic gap, and solve computational complexity Effect
- Summary
- Abstract
- Description
- Claims
- Application Information
AI Technical Summary
Problems solved by technology
Method used
Image
Examples
Embodiment 1
[0071] Embodiment 1: This embodiment is based on the target retrieval method of randomized visual lexicon group and contextual semantic information. First, aiming at the low efficiency of traditional clustering algorithms and the problem of synonymous and ambiguous visual words, E 2 LSH clusters the local feature points of the training image database to generate a set of randomized visual dictionaries that support dynamic expansion; secondly, select the query image and use a rectangular frame to define the target area, and then extract the query image and the image database according to Lowe's method SIFT features and perform E on them 2 LSH mapping realizes the matching of feature points and visual words; then, on the basis of the language model, uses the rectangular frame area and image saliency detection to calculate the retrieval score of each visual word in the query image and obtain the target model containing the target context semantic information ;Finally, for the pro...
Embodiment 2
[0073] Embodiment two: see figure 2 , image 3 , Figure 4 , the target retrieval method based on the randomized visual dictionary group and contextual semantic information of this embodiment adopts the following steps to generate 2 LSH's randomized visual dictionary set:
[0074] For each hash function g i (i=1,...,L), use it to perform hash mapping on the SIFT points of the training image library, and the points with very close distances in the space will be stored in the same bucket of the hash table, with the center of each bucket represents a visual word, each function g i can generate a hash table, a visual dictionary. Then, L functions g 1 ,..., g L can generate a visual dictionary group, the process is as follows figure 2 shown.
[0075] Among them, the detailed process of single visual dictionary generation can be described as follows:
[0076] (1) SIFT feature extraction of the training image library. In this paper, Oxford5K, a commonly used database for...
Embodiment 3
[0103] Embodiment 3: The difference between this embodiment and Embodiment 2 is that the following steps are used to measure the similarity:
[0104] The similarity between the query image q and any image d in the image database can be measured by the query likelihood p(q|d), then:
[0105] p ( q | d ) = Π i = 1 M q p ( q i | d ) - - - ( 14 )
[0106] Transforming this into a risk minimization problem, that is, given a query image q, the risk function for returning an image d is defined as follows:
[0107]
[0108]
[0109] p(θ D |d)p(r|θ Q ,θ D )dθ Q dθ D ...
PUM
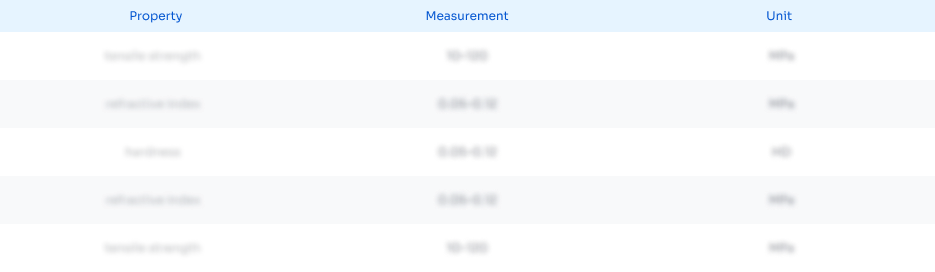
Abstract
Description
Claims
Application Information
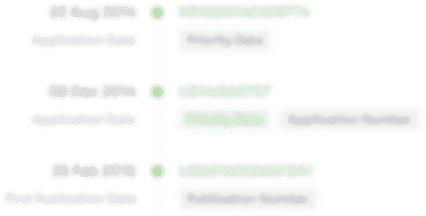
- R&D Engineer
- R&D Manager
- IP Professional
- Industry Leading Data Capabilities
- Powerful AI technology
- Patent DNA Extraction
Browse by: Latest US Patents, China's latest patents, Technical Efficacy Thesaurus, Application Domain, Technology Topic, Popular Technical Reports.
© 2024 PatSnap. All rights reserved.Legal|Privacy policy|Modern Slavery Act Transparency Statement|Sitemap|About US| Contact US: help@patsnap.com