Hyper-spectral remote sensing image semi-supervised classification method based on ground object class membership grading
A technology of hyperspectral remote sensing and classification methods, which is applied in the field of semi-supervised classification of hyperspectral remote sensing images, can solve problems such as the degree of diversity aggravated by multiple scattering effects, the phenomenon of different objects with the same spectrum, and classification difficulties, and achieve high-quality classification results. High classification accuracy, achieve the effect of fuzzy scoring
- Summary
- Abstract
- Description
- Claims
- Application Information
AI Technical Summary
Problems solved by technology
Method used
Image
Examples
Embodiment Construction
[0061] Below, take actual remote sensing image data as an example to illustrate the specific embodiment of the present invention:
[0062] The semi-supervised classification method based on membership score in the present invention is denoted by SCAS, and the two modes that adopt SLIC and cube over-segmentation are denoted by SCAS1 and SCAS2 respectively.
[0063] real data experiment
[0064] We test the performance of the proposed algorithm using an actual hyperspectral remote sensing image dataset. The data set is the Indian Pines data set taken in 1992 by the Airborne Visible / Infrared Imaging Spectrometer (AVIRIS). The dataset contains 145×145 pixels, 220 bands, the wavelength range is 0.4-2.5μm, and the spectral resolution is 10nm. After removing bands with low SNR or water absorption, the remaining 186 bands were used for algorithm validation. figure 1 A pseudo-color map of the image is shown, along with a map of the true distribution of features. According to the fi...
PUM
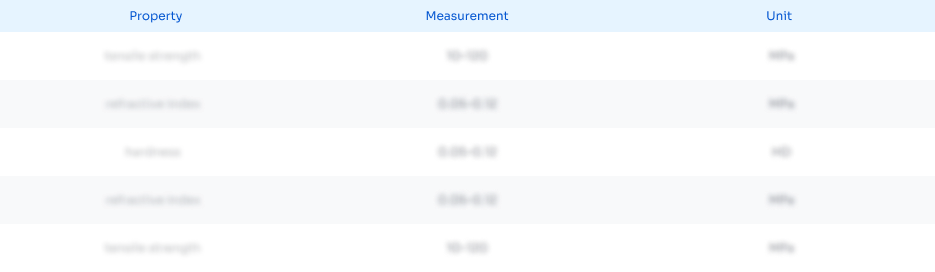
Abstract
Description
Claims
Application Information
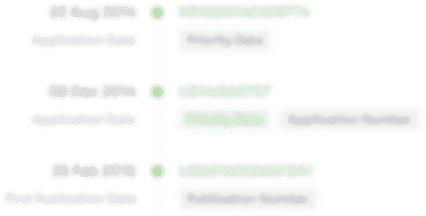
- R&D Engineer
- R&D Manager
- IP Professional
- Industry Leading Data Capabilities
- Powerful AI technology
- Patent DNA Extraction
Browse by: Latest US Patents, China's latest patents, Technical Efficacy Thesaurus, Application Domain, Technology Topic.
© 2024 PatSnap. All rights reserved.Legal|Privacy policy|Modern Slavery Act Transparency Statement|Sitemap