Fault diagnosis method for rolling bearing based on deep learning and SVM (Support Vector Machine)
A technology of support vector machines and rolling bearings, applied in computer parts, character and pattern recognition, instruments, etc., can solve the problems of poor interpretability of the reasoning process, the inability of neural networks to perform effective reasoning, and the inability to diagnose early characteristic bearings.
- Summary
- Abstract
- Description
- Claims
- Application Information
AI Technical Summary
Problems solved by technology
Method used
Image
Examples
Embodiment Construction
[0060] In order to overcome the deficiencies of the prior art, the present invention is based on the deep learning and support vector machine rolling bearing fault diagnosis method, firstly adopts the deep belief network to learn the essential characteristics of the training sample data, and then uses the support vector machine classification method to classify and identify the test samples , so as to determine the category of rolling bearing fault conditions, so as to improve the accuracy and effectiveness of rolling bearing fault diagnosis.
[0061] Deep Belief Network (DBN) has a powerful function expression ability, showing the excellent characteristics of learning the essential characteristics of data from a few samples. Studies have shown that the deep network structure composed of multiple nonlinear mapping layers is more effective than the shallow structure, and has good effects and efficiency in complex function representation and complex classification. The deep beli...
PUM
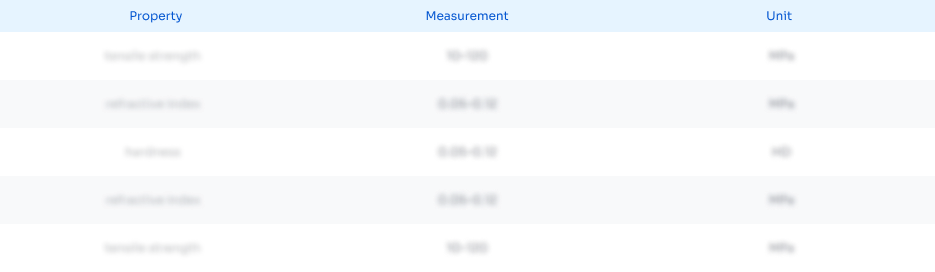
Abstract
Description
Claims
Application Information
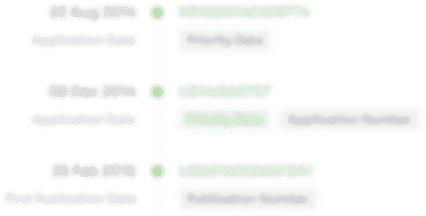
- R&D
- Intellectual Property
- Life Sciences
- Materials
- Tech Scout
- Unparalleled Data Quality
- Higher Quality Content
- 60% Fewer Hallucinations
Browse by: Latest US Patents, China's latest patents, Technical Efficacy Thesaurus, Application Domain, Technology Topic, Popular Technical Reports.
© 2025 PatSnap. All rights reserved.Legal|Privacy policy|Modern Slavery Act Transparency Statement|Sitemap|About US| Contact US: help@patsnap.com