Gaussian process regression soft measurement modeling method based on EGMM (Error Gaussian Mixture Model)
A technology of Gaussian process regression and modeling method, which is applied in the field of Gaussian process regression soft-sensor modeling and can solve problems such as complex modeling
- Summary
- Abstract
- Description
- Claims
- Application Information
AI Technical Summary
Problems solved by technology
Method used
Image
Examples
Embodiment Construction
[0014] Combine below figure 1 Shown, the present invention is described in further detail:
[0015] Taking the actual chemical process as an example, the sulfur recovery unit (Sulfur Recovery Unit, SRU) is used for sulfur-containing gases (mainly containing H 2 S, SO 2 ) to recover sulfur before it is discharged into the atmosphere, so as to prevent pollution to the environment, see figure 2 .
[0016] The SRU unit mainly deals with two kinds of acid gases: one is rich in H 2 S gas (also known as MEA gas); the other is the H-containing gas from the sulfur-containing sewage stripping equipment (SWS) 2 S, NH 3 The gas, also known as SWS gas. The main combustion chamber is used to process MEA gas, and it can be fully combusted in the case of sufficient air (AIR_MEA); the other combustion chamber is used to process SWS gas, and the incoming air flow rate can be written as AIR_SWS.
[0017] The descriptions of the 5 process variables and 2 leading variables are shown in Tab...
PUM
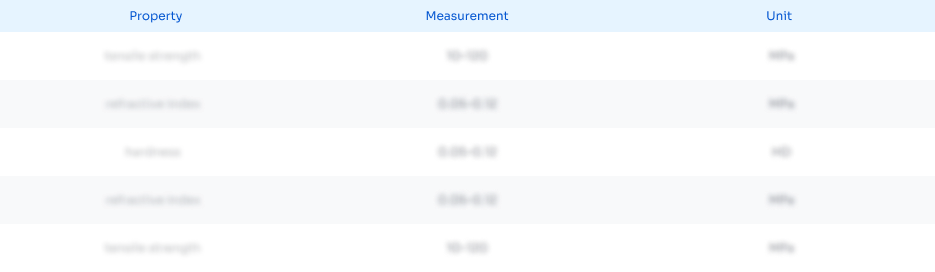
Abstract
Description
Claims
Application Information
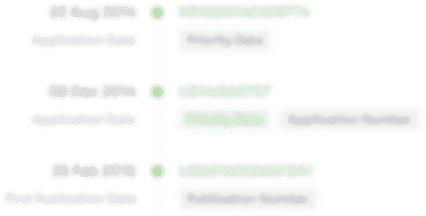
- R&D Engineer
- R&D Manager
- IP Professional
- Industry Leading Data Capabilities
- Powerful AI technology
- Patent DNA Extraction
Browse by: Latest US Patents, China's latest patents, Technical Efficacy Thesaurus, Application Domain, Technology Topic, Popular Technical Reports.
© 2024 PatSnap. All rights reserved.Legal|Privacy policy|Modern Slavery Act Transparency Statement|Sitemap|About US| Contact US: help@patsnap.com