Deep-level feature learning and watershed-based synthetic aperture radar (SAR) image classification method
A deep feature, watershed technology, applied in the field of image processing, to achieve the effect of improving regional consistency, enhancing robustness, and improving contrast
- Summary
- Abstract
- Description
- Claims
- Application Information
AI Technical Summary
Problems solved by technology
Method used
Image
Examples
Embodiment Construction
[0028] refer to figure 1 , the specific implementation steps of the present invention are as follows:
[0029] Step 1. Carry out watershed over-segmentation on the input SAR image to obtain the segmentation class label L={L 1 , L 2 ,...,L M}, where M is the total number of blocks divided by the watershed, L m is the class label of the mth sub-block, m∈[1,M].
[0030] Over-segmentation means that the value of M is much larger than the number of categories of the image, but it can guarantee that all pixels in each sub-block must belong to the same category of the image.
[0031] The specific process of this step is as follows:
[0032] (1a) Gamma (Gamma) filtering is performed on the input SAR image to obtain a smoothed image I;
[0033] (1b) Calculate the gradient map G of the classic edge detection operator (prewitt operator) of I 1 ;
[0034] (1c) for the gradient map G 1 Perform reconstruction to obtain the gradient map G 2 , the reconstruction rule is G 1 Set the t...
PUM
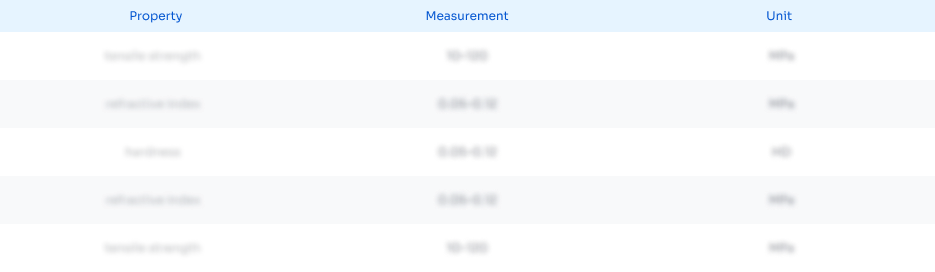
Abstract
Description
Claims
Application Information
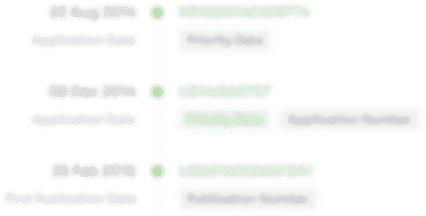
- R&D Engineer
- R&D Manager
- IP Professional
- Industry Leading Data Capabilities
- Powerful AI technology
- Patent DNA Extraction
Browse by: Latest US Patents, China's latest patents, Technical Efficacy Thesaurus, Application Domain, Technology Topic, Popular Technical Reports.
© 2024 PatSnap. All rights reserved.Legal|Privacy policy|Modern Slavery Act Transparency Statement|Sitemap|About US| Contact US: help@patsnap.com