Remote channel message compression method and system for electricity consumption collection system
A technology for collecting system and power consumption information, applied in transmission system, digital transmission system, adjusting channel coding and other directions, can solve the problems of data real-time cannot be guaranteed, consume a lot of time, transmission delay is large, etc., to reduce algorithm steps, The effect of high compression effect and low implementation cost
- Summary
- Abstract
- Description
- Claims
- Application Information
AI Technical Summary
Problems solved by technology
Method used
Image
Examples
Embodiment 1
[0026] Embodiment one , the present invention provides a communication message compression system used between the main station and the concentrator of the power consumption information collection system, such as figure 1 shown.
[0027] Probability model: a multi-layer context-dependent probability model is used in this method, which mainly has the following characteristics. For a certain character to be encoded in the message, the outline of the context tree gradually constructed by using the encoded string The information obtains the cumulative frequency of the character to be encoded in each order of context. There are two mechanisms for calculating the predicted probability of the character.
[0028] The first is a fallback mechanism, that is, to find out whether the character to be encoded appears in the current long context, and if so, output the cumulative frequency of the character to be encoded and the cumulative frequency of the previous character. If not presen...
Embodiment 2
[0033] Such as figure 2 with 3As shown, the present invention provides the communication environment of the method in the actual power consumption information collection system. For convenience of description, only the parts related to the example of the present invention are shown. The application communication environment of the present invention includes three parts, the concentrator, the remote communication channel, and the main station system. The concentrator is responsible for collecting and storing the power energy data and power quality data of the connected electric meters in a station area, and the master station system is responsible for sending various request data, setting parameters, and control commands to the concentrator according to actual business needs.
[0034] Because the characteristic of the remote channel of the power consumption information collection system is that the traffic volume of the uplink channel is much greater than the traffic volume o...
Embodiment 3
[0037] In the embodiment of the present invention, the data to be compressed is compressed online, that is, the message data can be compressed while being generated, without waiting for all the message data to be generated before being compressed, which reduces the transmission delay of the data inside the concentrator.
[0038] Such as Figure 4 As shown, the present invention provides a schematic flow chart of a specific data compression algorithm, which is described in detail as follows:
[0039] Step S1, initialize the search tree, and set the root node to be empty.
[0040] Step S2, obtain the next character S to be encoded.
[0041] Steps S3-S6, judge whether S is an escape character (whether the current context node has child nodes, if so, output an escape character, add the characters appearing in the current context to the excluded character set, and turn to the next shorter context Node, execute S3~S6 in a loop until it is not an escape character.
[0042] Step S7...
PUM
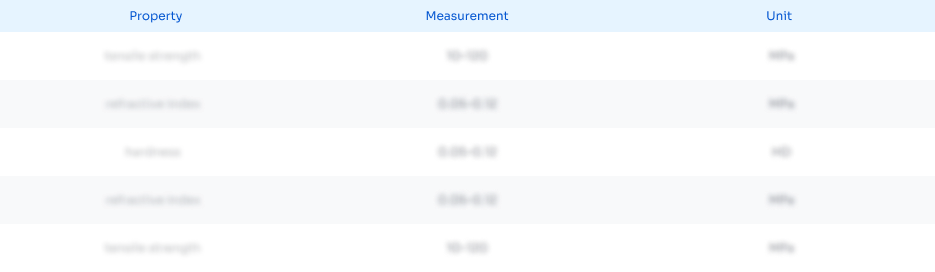
Abstract
Description
Claims
Application Information
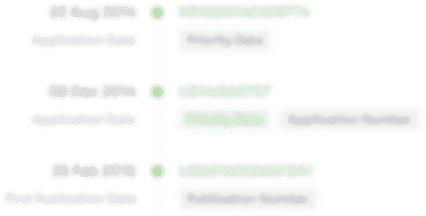
- Generate Ideas
- Intellectual Property
- Life Sciences
- Materials
- Tech Scout
- Unparalleled Data Quality
- Higher Quality Content
- 60% Fewer Hallucinations
Browse by: Latest US Patents, China's latest patents, Technical Efficacy Thesaurus, Application Domain, Technology Topic, Popular Technical Reports.
© 2025 PatSnap. All rights reserved.Legal|Privacy policy|Modern Slavery Act Transparency Statement|Sitemap|About US| Contact US: help@patsnap.com