Bearing fault diagnosis method based on multi-feature manifold learning and support vector machine
A technology of support vector machine and manifold learning, which is applied in the field of mechanical fault diagnosis and computer artificial intelligence, and can solve problems such as inability to diagnose early feature bearings of faults, large amount of calculation, and poor interpretability of reasoning process.
- Summary
- Abstract
- Description
- Claims
- Application Information
AI Technical Summary
Problems solved by technology
Method used
Image
Examples
Embodiment Construction
[0086] The present invention will be described in further detail below in conjunction with the accompanying drawings.
[0087] The idea of the rolling bearing fault diagnosis method based on multi-feature manifold learning and support vector machine in the present invention is to first obtain the time-domain features, frequency-domain features, and time-frequency domain features of the mechanical rolling bearing data to obtain the feature matrix; then use the manifold learning algorithm Extract the hidden low-dimensional flow components from the high-dimensional data feature set, effectively eliminate redundancy, and extract the inherent essential characteristics of the original signal; finally, use the support vector machine classification method to classify and identify the test samples to determine the category of rolling bearing fault conditions , to realize the diagnosis of rolling bearing fault categories, so as to improve the accuracy and effectiveness of rolling beari...
PUM
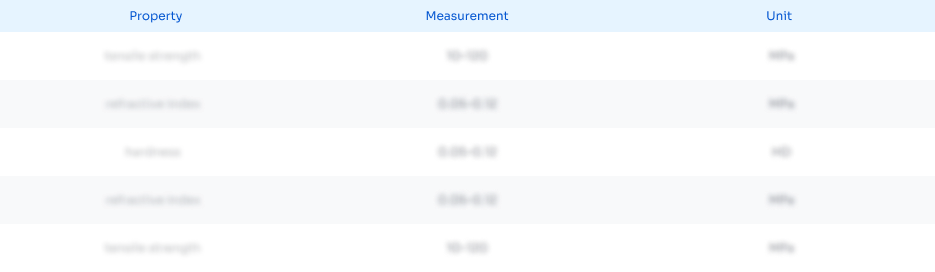
Abstract
Description
Claims
Application Information
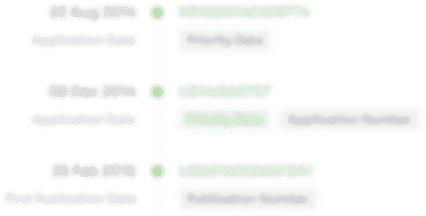
- R&D Engineer
- R&D Manager
- IP Professional
- Industry Leading Data Capabilities
- Powerful AI technology
- Patent DNA Extraction
Browse by: Latest US Patents, China's latest patents, Technical Efficacy Thesaurus, Application Domain, Technology Topic, Popular Technical Reports.
© 2024 PatSnap. All rights reserved.Legal|Privacy policy|Modern Slavery Act Transparency Statement|Sitemap|About US| Contact US: help@patsnap.com