Polarized SAR (Synthetic Aperture Radar) image semi-supervised classification method capable of considering characteristic optimization
A feature optimization and classification method technology, which is applied in the field of remote sensing image processing, can solve the problems of error accumulation, large difference in clustering effect, and increased calculation burden, and achieve the effects of improving classification accuracy, avoiding error accumulation, and avoiding coherence
- Summary
- Abstract
- Description
- Claims
- Application Information
AI Technical Summary
Problems solved by technology
Method used
Image
Examples
Embodiment Construction
[0043] The technical solutions of the present invention will be described in detail below in conjunction with the accompanying drawings.
[0044] Such as figure 1 As shown, the present invention provides a kind of polarization SAR image semi-supervised classification method that considers feature optimization, comprises the following steps:
[0045] (1) Read in a polarimetric SAR image to be classified, and preprocess it, specifically, use the refined polarization LEE (Refined Lee) filter algorithm to filter the SAR image to be classified, and the sliding window size of this algorithm is 7 *7 pixels, so as to remove the speckle noise, eliminate the influence of the speckle noise, obtain the polarimetric SAR image after denoising, and enhance the readability of the image;
[0046] (2) Perform feature extraction on the denoised polarimetric SAR image, the extracted features are shown in Table 1.
[0047] Table 1
[0048]
[0049] When performing feature extraction, various...
PUM
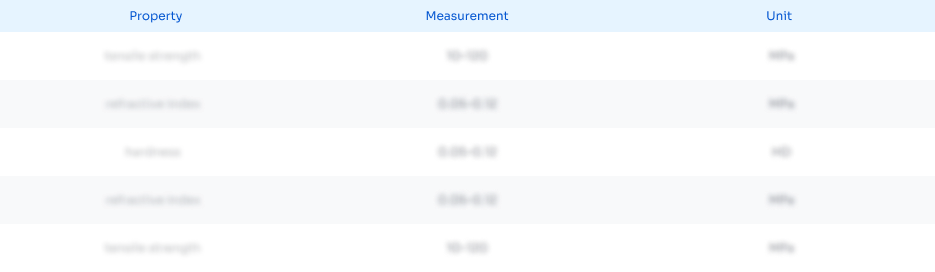
Abstract
Description
Claims
Application Information
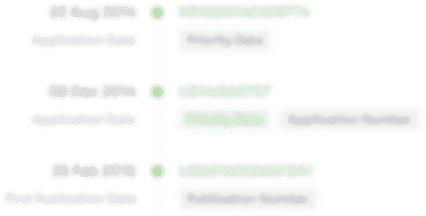
- Generate Ideas
- Intellectual Property
- Life Sciences
- Materials
- Tech Scout
- Unparalleled Data Quality
- Higher Quality Content
- 60% Fewer Hallucinations
Browse by: Latest US Patents, China's latest patents, Technical Efficacy Thesaurus, Application Domain, Technology Topic, Popular Technical Reports.
© 2025 PatSnap. All rights reserved.Legal|Privacy policy|Modern Slavery Act Transparency Statement|Sitemap|About US| Contact US: help@patsnap.com