Least square support vector machine-based fluctuating wind velocity prediction method
A technology of support vector machine and pulsating wind speed, which can be used in forecasting, computer parts, instruments, etc., and can solve the problems of time-consuming and low simulation accuracy of support vector machine.
- Summary
- Abstract
- Description
- Claims
- Application Information
AI Technical Summary
Problems solved by technology
Method used
Image
Examples
Embodiment 1
[0094] see figure 1 , a fluctuating wind speed prediction method based on the least squares support vector machine, the specific steps are as follows:
[0095] 1) Select a super high-rise building and determine the parameters required for numerical simulation of fluctuating wind speed: the simulated building height and the heights of the simulated wind speed points, the average wind speed at a height of 10 meters, surface roughness coefficient, ground roughness index, simulation correlation function, a set number of fluctuating wind speed time histories uniformly distributed along the height generated by the numerical simulation of the ARMA method, as the limited original fluctuating wind speed sample data;
[0096] 2) Use the PSO algorithm to determine the parameters. Determine the population size and evolution times, set c 1 and c 2 、w max and w min The value of r is randomly generated 1 and r 2 ; According to the preliminary range of model parameters obtained by cros...
Embodiment 2
[0100] This is based on the fluctuating wind speed prediction method of the LSSVM model using the B-RBF combined kernel function. The specific steps are as follows:
[0101] The first step is to select a super high-rise building with a height of 150 meters in a city center, and take points every 10 meters along the height direction as the simulated wind speed points. Other relevant parameters are shown in Table 1:
[0102] Table 1 Related simulation parameters
[0103]
[0104] Indicates the average wind speed at a height of 10m.
[0105] In the second step, a certain number of fluctuating wind speed time histories uniformly distributed along the height generated by the ARMA numerical simulation are used as limited original fluctuating wind speed sample data.
[0106] In order to verify the validity of the prediction based on the machine learning method, a part of the sample data set needs to be used for machine learning, and another part of the sample data set is used ...
PUM
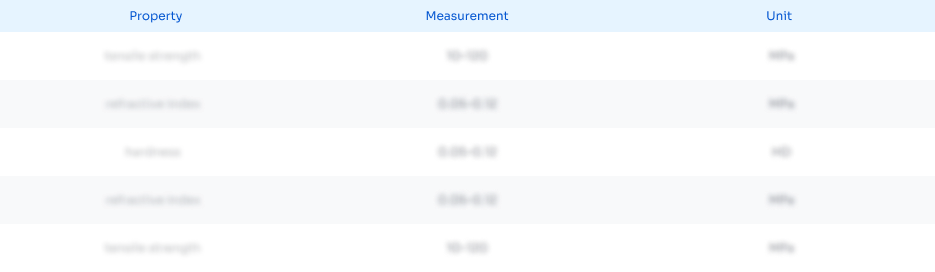
Abstract
Description
Claims
Application Information
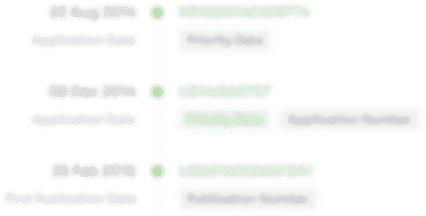
- R&D Engineer
- R&D Manager
- IP Professional
- Industry Leading Data Capabilities
- Powerful AI technology
- Patent DNA Extraction
Browse by: Latest US Patents, China's latest patents, Technical Efficacy Thesaurus, Application Domain, Technology Topic, Popular Technical Reports.
© 2024 PatSnap. All rights reserved.Legal|Privacy policy|Modern Slavery Act Transparency Statement|Sitemap|About US| Contact US: help@patsnap.com