Excitation surge and fault current identification method based on kernel function limit learning machine
An extreme learning machine, fault current technology, applied in character and pattern recognition, machine learning, electrical components and other directions, can solve the problems of accuracy limitation, high requirement for the number of training samples, energy leakage of wavelet decomposition, etc. The effect of stable generalization performance and short training time
- Summary
- Abstract
- Description
- Claims
- Application Information
AI Technical Summary
Problems solved by technology
Method used
Image
Examples
Embodiment
[0058] In order to verify the effect and reliability of the method of the present invention, the following example verification is carried out. The test simulation is carried out on the EMTDC electromagnetic transient simulation test platform. The test simulation transformer is a three single-phase transformer group, and the high and low voltage sides are Y / D connection. The specific parameters of the single-phase transformer are as follows: rated capacity 10500kVA; low-voltage side rated voltage 10.5kV; low-voltage side rated current 1000A; high-voltage side rated voltage 110kV; high-voltage side rated current 95.45A; no-load current is 0.14%; no-load loss is 6.8kW ; The short-circuit loss is 7.73kW; the short-circuit voltage is 10.02%. Test conditions: no-load closing, single-phase grounding fault (short-circuit at 32% of phase A), two-phase grounding short-circuit fault (short-circuit at 32% of phase A and B), three-phase short-circuit (A, B and C) Short circuit at 32% of ...
PUM
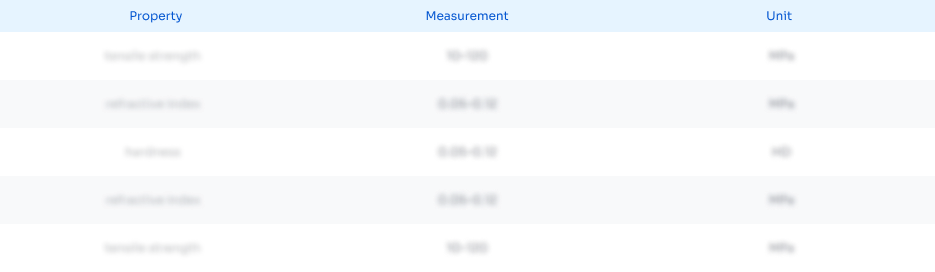
Abstract
Description
Claims
Application Information
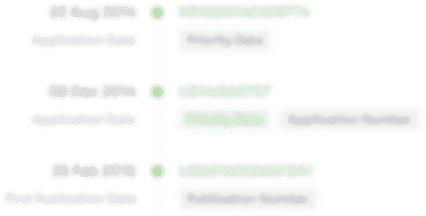
- R&D Engineer
- R&D Manager
- IP Professional
- Industry Leading Data Capabilities
- Powerful AI technology
- Patent DNA Extraction
Browse by: Latest US Patents, China's latest patents, Technical Efficacy Thesaurus, Application Domain, Technology Topic, Popular Technical Reports.
© 2024 PatSnap. All rights reserved.Legal|Privacy policy|Modern Slavery Act Transparency Statement|Sitemap|About US| Contact US: help@patsnap.com