Nonlinear process fault detection method based on differential locality preserving projection (DLPP)
It is a technology of partial maintenance projection and fault detection. It is applied in the direction of program control, electrical program control, and comprehensive factory control. It can solve the problem of loss, failure to find hidden internal useful information, and data manifold between data cannot be maintained. problem, to achieve good detection results and improve the effect of fault detection
- Summary
- Abstract
- Description
- Claims
- Application Information
AI Technical Summary
Problems solved by technology
Method used
Image
Examples
Embodiment Construction
[0015] The present invention will be described in detail below in conjunction with examples.
[0016] The present invention preprocesses (expands into two-dimensional and standardized) a large amount of normal historical data, uses a difference algorithm, eliminates the nonlinear structure of the data, and multiplies the distance between the two samples after projection by the corresponding weight. value is the smallest, find the projection matrix A , the control limits of the SPE statistic are calculated by kernel density estimation. The difference operation is performed on the new samples and projected to the low-dimensional space, and the SPE statistics are calculated for fault detection. The technology solves the problem that the traditional algorithm cannot eliminate the non-linearity well while maintaining the internal structure of the data when it is used for the fault diagnosis of the non-linear process. In order to better detect faults in nonlinear processes, it is ...
PUM
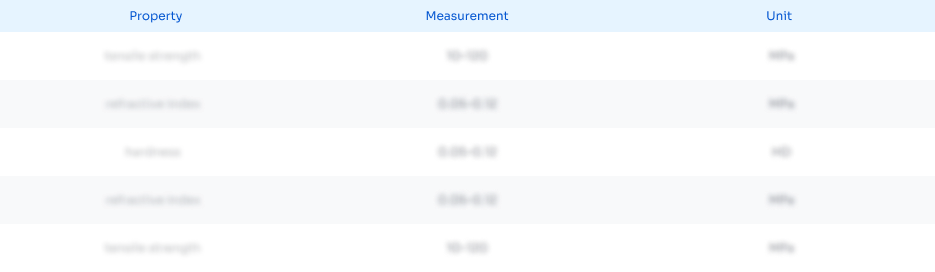
Abstract
Description
Claims
Application Information
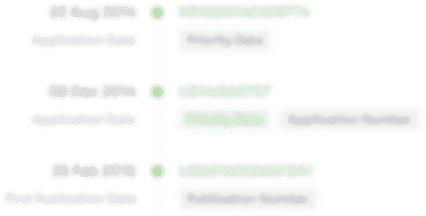
- R&D Engineer
- R&D Manager
- IP Professional
- Industry Leading Data Capabilities
- Powerful AI technology
- Patent DNA Extraction
Browse by: Latest US Patents, China's latest patents, Technical Efficacy Thesaurus, Application Domain, Technology Topic, Popular Technical Reports.
© 2024 PatSnap. All rights reserved.Legal|Privacy policy|Modern Slavery Act Transparency Statement|Sitemap|About US| Contact US: help@patsnap.com