Multi-species feature selection and unknown gene identification methods
A feature selection and multi-species technology, applied in the field of life sciences, can solve the problems of lack of comprehensiveness in the regulation of non-coding RNA expression and lack of identification standards for identifying non-coding RNAs
- Summary
- Abstract
- Description
- Claims
- Application Information
AI Technical Summary
Problems solved by technology
Method used
Image
Examples
Embodiment 1
[0076] For each species (diagonal element positions), non-coding RNAs are well distinguished from defined protein-coding, untranslated, and negative control regions. In order to verify the robustness of the common features selected by RNAfeature, the accuracy of its cross-species prediction was tested.
[0077] Such as image 3 As shown, the accuracy of cross-species prediction of non-coding RNA, measuring the accuracy of four species (H for human, M for mouse, F for Drosophila, W for nematode) models to distinguish four types of genomic elements (accuracy ACC) . The accuracy of within-species predictions is shown on the diagonal, and the accuracy of cross-species predictions is shown as off-diagonal elements. In the figure, red represents the identified protein coding region (CDS), purple represents the 5' and 3' untranslated regions (UTRs), green represents canonical non-coding RNAs (canonical ncRNAs), and blue represents the negative control region (negative control .
...
Embodiment 2
[0082] RNAfeature only uses classic non-coding RNAs (including rRNA, tRNA, snRNA, miRNA, Y RNA, etc.) as positive sample sets, so it is necessary to test the ability of common features to predict new non-coding RNAs. For this reason, the present invention designs cross-validation across categories, that is, firstly a certain type of non-coding RNA (including rRNA, tRNA, snRNA, miRNA, Y RNA, etc.) is removed from the RNAfeature training set, and then the common feature training model is used , use the model to predict the type of non-coding RNA that will be eliminated.
[0083] Such as Figure 4 As shown, using the human data as an example, the boxplot shows the probability distribution (y-axis) when a specific type of genomic element (title of each window) is predicted to be different classes (x-axis). Different colors represent different classes: red for defined protein coding regions (CDS), purple for 5' and 3' untranslated regions (UTRs), green for canonical ncRNAs, and bl...
Embodiment 3
[0086] Cross-species cross-validation was also performed for the remaining species. Such as Figure 5 As shown, in the data of 4 species (human, mouse, Drosophila and C. Accuracy of coding RNAs (canonical ncRNAs) and negative control regions. NA indicates that this type of non-coding RNA is not present in this species. Darker colors represent higher accuracy.
[0087] The results showed that the accuracy of the cross-species cross-validation for the four species was high, and it was much higher than the accuracy of random guessing. In comparison, the accuracy of the human and mouse models is higher than that of the Drosophila and nematode models, which may be due to the greater number of annotated non-coding RNAs in humans and mice, and the model training is more difficult. full.
PUM
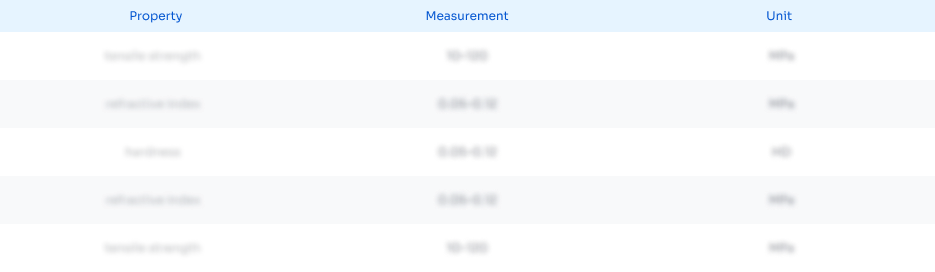
Abstract
Description
Claims
Application Information
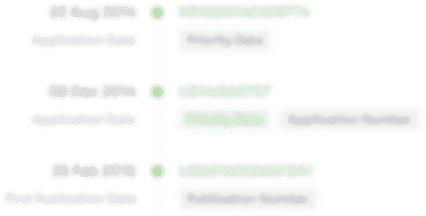
- Generate Ideas
- Intellectual Property
- Life Sciences
- Materials
- Tech Scout
- Unparalleled Data Quality
- Higher Quality Content
- 60% Fewer Hallucinations
Browse by: Latest US Patents, China's latest patents, Technical Efficacy Thesaurus, Application Domain, Technology Topic, Popular Technical Reports.
© 2025 PatSnap. All rights reserved.Legal|Privacy policy|Modern Slavery Act Transparency Statement|Sitemap|About US| Contact US: help@patsnap.com