A Segmentation Method of Cranial Magnetic Resonance Image Based on Spatial Mixture Model
A magnetic resonance image and space mixing technology, applied in image analysis, image enhancement, image data processing, etc., can solve the problems of noise influence, large amount of calculation, and large algorithm complexity, etc., and achieve strong noise resistance and accurate model solution Efficient effect
- Summary
- Abstract
- Description
- Claims
- Application Information
AI Technical Summary
Problems solved by technology
Method used
Image
Examples
Embodiment Construction
[0033] Such as figure 1 As shown, a brain MRI image segmentation method based on the spatial mixture model, the steps are as follows:
[0034] Step 101, performing a preprocessing operation on the original brain magnetic resonance image to be segmented: using the watershed algorithm to remove non-brain tissue, and obtaining a preprocessed image with N rows and M columns;
[0035] Step 102, converting the preprocessed image into a row vector;
[0036] Specifically, follow the steps below to convert the preprocessed image into a row vector:
[0037] First read the preprocessed image matrix in the form of columns to obtain an N*M-dimensional column vector;
[0038] Transpose an N*M-dimensional column vector into a corresponding row vector.
[0039] Step 103, using the K-means method to initially cluster the row vectors, and setting the number of clusters K=3, respectively representing gray matter, white matter and cerebrospinal fluid;
[0040] Step 104, building a model for t...
PUM
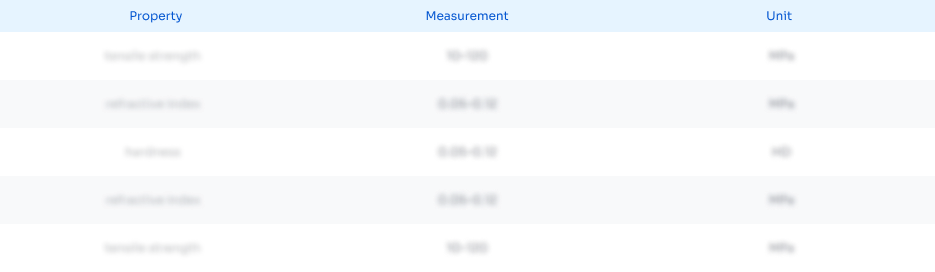
Abstract
Description
Claims
Application Information
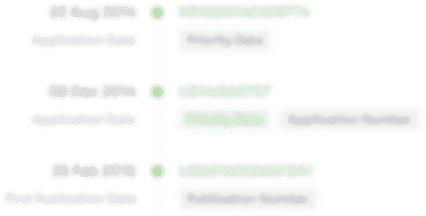
- R&D
- Intellectual Property
- Life Sciences
- Materials
- Tech Scout
- Unparalleled Data Quality
- Higher Quality Content
- 60% Fewer Hallucinations
Browse by: Latest US Patents, China's latest patents, Technical Efficacy Thesaurus, Application Domain, Technology Topic, Popular Technical Reports.
© 2025 PatSnap. All rights reserved.Legal|Privacy policy|Modern Slavery Act Transparency Statement|Sitemap|About US| Contact US: help@patsnap.com