Diesel vehicle tail gas smoke intensity detection method based on deep residual error learning network
A technology for learning networks and detection methods, applied in the field of image processing, can solve the problems of insufficient accuracy of deep residual learning networks, inaccurate detection, and high cost, so as to avoid cheating, strengthen generalization capabilities, and save manpower. physical effect
- Summary
- Abstract
- Description
- Claims
- Application Information
AI Technical Summary
Problems solved by technology
Method used
Image
Examples
Embodiment Construction
[0030] Such as figure 1 Shown, the specific implementation steps of the present invention are as follows:
[0031] 1. Set up CCD high-speed cameras on both sides of the road.
[0032] 1.1) The CCD camera records the diesel vehicle exhaust video screen. It transmits the reflected light of the subject to the lens, and then focuses it on the CCD chip through the lens. The CCD accumulates a corresponding proportion of charge according to the intensity of the light. The charge accumulated by each pixel moves outward under the control of the timing of the screen. After filtering, After amplifying processing, a video signal output is formed. Connect the video signal to the video input of the monitor and you can see the same video image as the original image.
[0033] 1.2) Use the image acquisition card to collect the image information into the computer. The video images recorded by the CCD camera can be transmitted to the computer through the image acquisition card, and these ima...
PUM
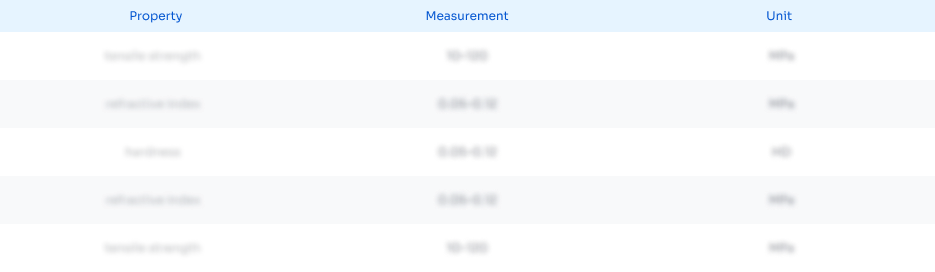
Abstract
Description
Claims
Application Information
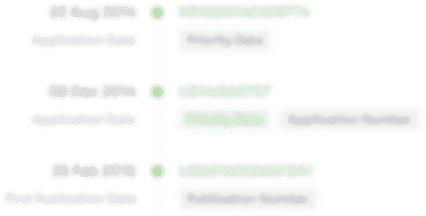
- R&D Engineer
- R&D Manager
- IP Professional
- Industry Leading Data Capabilities
- Powerful AI technology
- Patent DNA Extraction
Browse by: Latest US Patents, China's latest patents, Technical Efficacy Thesaurus, Application Domain, Technology Topic.
© 2024 PatSnap. All rights reserved.Legal|Privacy policy|Modern Slavery Act Transparency Statement|Sitemap