Retinal vessel segmentation method based on combination of deep learning and traditional method
A retinal blood vessel and deep learning technology, applied in the field of retinal blood vessel segmentation, can solve problems such as low calculation efficiency, classification failure, and large storage overhead, and achieve the goals of improving robustness and accuracy, wide applicability, and reducing feature redundancy Effect
- Summary
- Abstract
- Description
- Claims
- Application Information
AI Technical Summary
Problems solved by technology
Method used
Image
Examples
Embodiment Construction
[0040] Describe in detail below in conjunction with accompanying drawing:
[0041] The technical block diagram of the present invention is as figure 1 shown. The specific implementation steps are as follows:
[0042] 1. Pretreatment
[0043] The same preprocessing is performed on each retinal fundus image whether it is a training set or a test set.
[0044] 1) Extract the green channel with relatively high contrast in the RGB three channels of the color retinal image. Secondly, due to problems such as shooting angles, the brightness of the collected retinal fundus images is often uneven, or the lesion area is difficult to distinguish from the background due to problems such as too bright or too dark and low contrast in the image. Therefore, we Perform normalization processing, and then perform CLAHE processing on the normalized retinal image to improve the quality of the retinal fundus image, balance the brightness of the fundus image, and make it more suitable for subsequ...
PUM
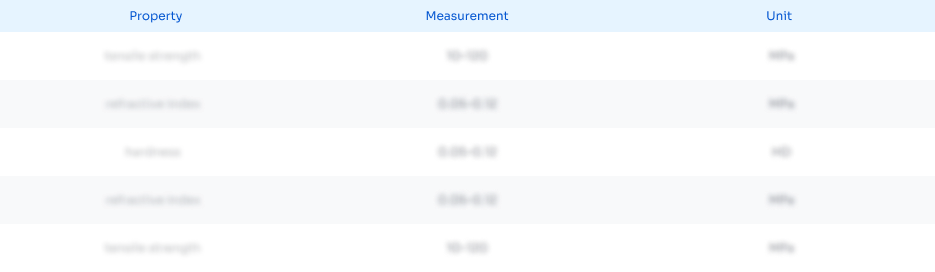
Abstract
Description
Claims
Application Information
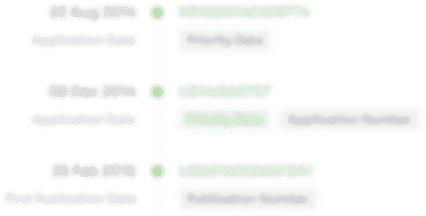
- R&D Engineer
- R&D Manager
- IP Professional
- Industry Leading Data Capabilities
- Powerful AI technology
- Patent DNA Extraction
Browse by: Latest US Patents, China's latest patents, Technical Efficacy Thesaurus, Application Domain, Technology Topic, Popular Technical Reports.
© 2024 PatSnap. All rights reserved.Legal|Privacy policy|Modern Slavery Act Transparency Statement|Sitemap|About US| Contact US: help@patsnap.com