Selective integrated learning-based rotating machinery fault diagnosis method
An integrated learning and fault diagnosis technology, applied in neural learning methods, complex mathematical operations, computer components, etc., can solve problems such as data complexity of rotating machinery
- Summary
- Abstract
- Description
- Claims
- Application Information
AI Technical Summary
Problems solved by technology
Method used
Image
Examples
Embodiment Construction
[0043] 1. Generation of base classifier
[0044]The present invention adopts probabilistic neural networks (PNN) as a base classifier to realize the recognition of the fault mode of the rotating machinery. PNN is a forward neural network based on Bayesian minimum risk criterion and nuclear Fisher discriminant, which was invented by D.F.Specht in 1990. Usually, the PNN network structure is as figure 1 shown.
[0045] exist figure 1 In, we can find that the PNN network can usually be divided into four layers: the input layer, the model layer, the accumulation layer and the output layer. When a test input signal is given to the network, the input layer will first calculate the distance between the data point in the signal and the training vector point; then, the pattern layer will use a radial basis function to convert the distance into Corresponding weight parameters; in the overlay layer, the weight parameters of each point in the input data for different failure modes will...
PUM
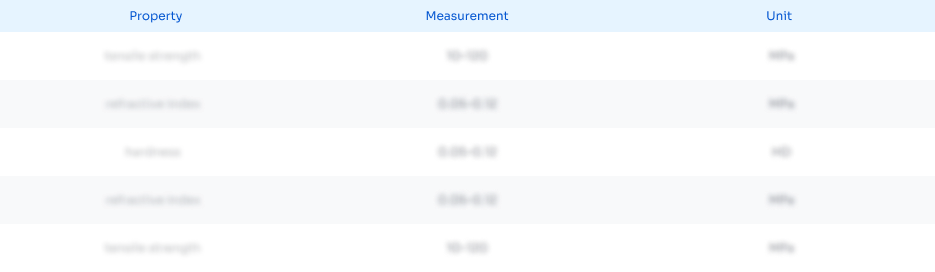
Abstract
Description
Claims
Application Information
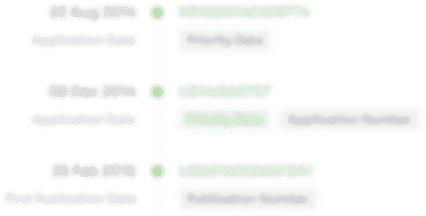
- R&D Engineer
- R&D Manager
- IP Professional
- Industry Leading Data Capabilities
- Powerful AI technology
- Patent DNA Extraction
Browse by: Latest US Patents, China's latest patents, Technical Efficacy Thesaurus, Application Domain, Technology Topic.
© 2024 PatSnap. All rights reserved.Legal|Privacy policy|Modern Slavery Act Transparency Statement|Sitemap