Trajectory control method and control system of underwater robot based on deep reinforcement learning
An underwater robot, reinforcement learning technology, applied in general control systems, control/regulation systems, height or depth control, etc., can solve problems such as low trajectory tracking accuracy
- Summary
- Abstract
- Description
- Claims
- Application Information
AI Technical Summary
Problems solved by technology
Method used
Image
Examples
Embodiment 1
[0155] This embodiment discloses a trajectory control method for an underwater robot based on deep reinforcement learning, which is characterized in that it includes a learning phase and an application phase; in the learning phase, the operating process of the underwater robot is simulated by a simulator, and the simulated data is collected. According to the data of the operating underwater robot simulated by the device, the decision-making neural network, auxiliary decision-making neural network, evaluation neural network and auxiliary evaluation neural network are studied according to these data; the specific steps are as follows:
[0156] S1. First, four neural networks are established, which are respectively used as decision-making neural network, auxiliary decision-making neural network, evaluation neural network and auxiliary evaluation neural network, and the neural network parameters of the four neural networks are initialized; the parameters of the neural network refer ...
Embodiment 2
[0221] This embodiment discloses a trajectory control method based on an underwater robot. The difference between it and the trajectory control method based on an underwater robot disclosed in Embodiment 1 is that the learning stage in this embodiment also includes the following steps: S8. During the operation of the robot, real-time data are collected at each moment, and the following re-learning is carried out for the decision-making neural network, auxiliary decision-making neural network, evaluation neural network and auxiliary evaluation neural network learned in step S7, specifically:
[0222] S81. Initialize the experience data buffer first; use the decision-making neural network, auxiliary decision-making neural network, evaluation neural network, and auxiliary evaluation neural network that have been learned in step S7 as the initial neural network; then, for the above-mentioned initial neural network, start from the initial moment , enter step S82 to start learning; ...
Embodiment 3
[0251] This embodiment discloses a trajectory control method for an underwater robot based on deep reinforcement learning, which is characterized in that it includes a learning phase and an application phase; in the learning phase, the specific steps are as follows:
[0252] S1. First, four neural networks are established, which are respectively used as decision-making neural network, auxiliary decision-making neural network, evaluation neural network and auxiliary evaluation neural network, and the neural network parameters of the four neural networks are initialized; the parameters of the neural network refer to The connection weights of each layer of neurons in the neural network; at the same time, an experience data buffer is established and initialized; then, for the above-mentioned four initialized neural networks, from the initial moment, enter step S2 to start learning;
[0253] S2, judging whether the current moment is the initial moment;
[0254] If so, then collect ...
PUM
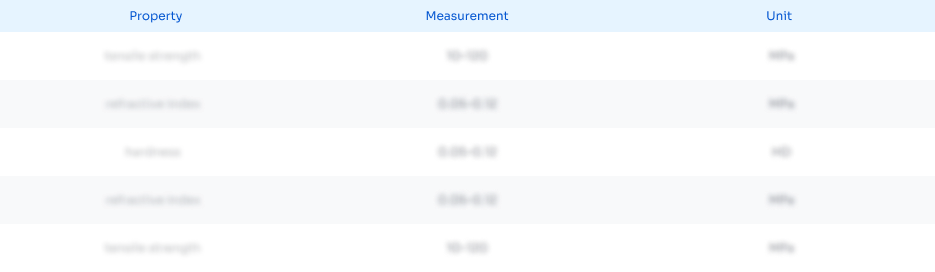
Abstract
Description
Claims
Application Information
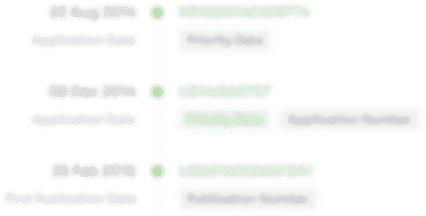
- R&D Engineer
- R&D Manager
- IP Professional
- Industry Leading Data Capabilities
- Powerful AI technology
- Patent DNA Extraction
Browse by: Latest US Patents, China's latest patents, Technical Efficacy Thesaurus, Application Domain, Technology Topic, Popular Technical Reports.
© 2024 PatSnap. All rights reserved.Legal|Privacy policy|Modern Slavery Act Transparency Statement|Sitemap|About US| Contact US: help@patsnap.com