Deep convolutional neural network based SAR image sea ice sorting method
A deep convolution, neural network technology, applied in the field of sea ice monitoring, to achieve the effect of strong operability, meeting real-time requirements, and short processing time
- Summary
- Abstract
- Description
- Claims
- Application Information
AI Technical Summary
Problems solved by technology
Method used
Image
Examples
Embodiment 1
[0100] refer to figure 1 , figure 1 It is a schematic diagram of the sea ice classification method based on deep convolutional neural network in SAR images. A kind of SAR image sea ice classification method based on depth convolutional neural network of the present invention comprises the following steps:
[0101] S01: Segment existing sea ice SAR images
[0102] S011: Segment existing sea ice SAR images into regional maps of different sea ice types;
[0103] S012: Cut the area map into multiple sample sizes of different sizes, and use them as original training samples;
[0104] S02: Perform data preprocessing
[0105] S021: Labeling the original training samples;
[0106] S022: Convert data storage format;
[0107] S023: data averaging processing;
[0108] S03: Conduct model training and build a model
[0109] S031: Construct a convolutional neural network model (CNN model);
[0110] S032: Divide the sample data into a training set and a test set;
[0111] S033: Sen...
Embodiment 2
[0123] A kind of SAR image sea ice classification method based on deep convolutional neural network of the present invention, its specific working procedure is:
[0124] S01: Segment existing sea ice SAR images
[0125] S011: Segment the existing sea ice SAR images into regional maps of different sea ice types according to the interpretation map (Ice Chart) marked by experts;
[0126] S012: Cut the area map into multiple sample sizes of different sizes, and use them as original training samples;
[0127] S02: Perform data preprocessing
[0128] S021: Labeling the original training samples;
[0129] S022: Convert data storage format;
[0130] S023: data averaging processing;
[0131] S03: Conduct model training and build a model
[0132] S031: Construct a convolutional neural network model;
[0133] S032: Divide the sample data into a training set and a test set according to different sizes;
[0134] S033: Send the training set and the test set to the convolutional neura...
Embodiment 3
[0175] refer to figure 2 , figure 2 is the process of convolutional neural network. A specific implementation of the convolutional neural network model of the SAR image sea ice classification method based on the deep convolutional neural network of the present invention is as follows:
[0176] A01: Construct a convolutional neural network model, including 3 convolutional layers, 3 pooling layers, 1 fully connected layer, and Softmax loss layer;
[0177] Its parameters are as follows:
[0178] The convolution kernel size of the convolution layer is 5×5, the convolution kernel quantity of the first convolution layer is 32, the convolution kernel quantity of the second convolution layer is 32, and the convolution kernel quantity of the third convolution layer is 32. The number of convolution kernels in the product layer is 64;
[0179] The pooling layer filter is 3×3, and the step size is 2;
[0180] The activation function is a ReLu function;
[0181] The learning rate η...
PUM
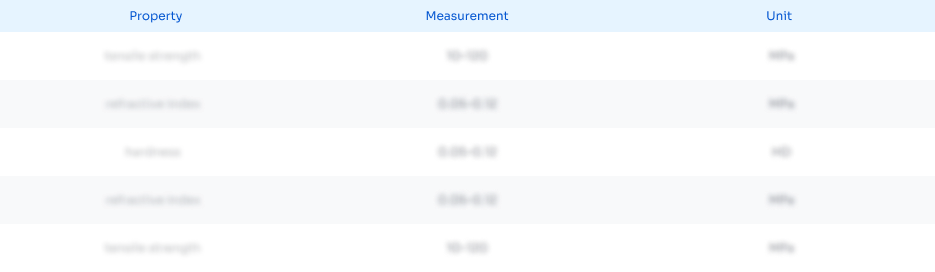
Abstract
Description
Claims
Application Information
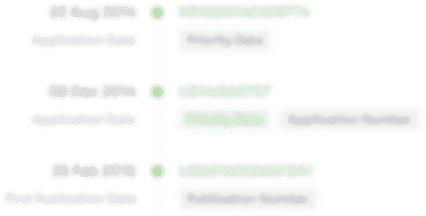
- R&D
- Intellectual Property
- Life Sciences
- Materials
- Tech Scout
- Unparalleled Data Quality
- Higher Quality Content
- 60% Fewer Hallucinations
Browse by: Latest US Patents, China's latest patents, Technical Efficacy Thesaurus, Application Domain, Technology Topic, Popular Technical Reports.
© 2025 PatSnap. All rights reserved.Legal|Privacy policy|Modern Slavery Act Transparency Statement|Sitemap|About US| Contact US: help@patsnap.com