Methods of predicting pathogenicity of genetic sequence variants
A gene sequence and pathogenicity technology, applied in the field of predicting the pathogenicity of gene sequences, can solve problems such as time-consuming
- Summary
- Abstract
- Description
- Claims
- Application Information
AI Technical Summary
Problems solved by technology
Method used
Image
Examples
example 1
[0120] Example 1: Training data, training a machine learning model, and testing the trained machine learning model
[0121] Figure 5A An exemplary embodiment of the present invention is illustrated. At an electronic device having at least one processor and memory, a machine learning model is trained based on the training data. The training data includes a labeled benign gene sequence variant dataset and an unlabeled gene sequence variant dataset. like Figure 5A As shown in , the labeled benign dataset was obtained from the 1000 Genomes Project by filtering the database for gene sequence variants with greater than 95% derived allele frequency (DAF), which were assumed to be benign due to their high frequency . The labeled benign dataset has 881,924 gene sequence variants. The unlabeled gene sequence variation dataset was simulated using CADD's variation simulation software, which mutates loci according to local mutation rates in sliding 1.1 Mb windows. Mutation rates we...
example 2
[0123] Example 2: Semi-supervised clustering of mutant machine learning models compared to previous methods
[0124] The methods described in this paper perform better than previously known methods in predicting the pathogenicity of sequence variants. One embodiment of the method described herein (in Figure 6A , Figure 6B , Figure 7A , Figure 7B , Figure 8 and Figure 10 labelled in and described herein as "SSCM-Pathogenic") manifestations including CADD (described in Kircher et al. Nature Genetics, 46(3):310-5 (2014)) and other known methods A comparison of known methods for generating gene sequence variant pathogenicity scores.
[0125] As proof of conception of one embodiment of the method described herein, a genetic sequence variation test dataset is classified into pathogenic clusters and benign clusters. The genome sequence variation test dataset includes the known pathogenic gene sequence variation test dataset and the known benign gene sequence variation te...
example 3
[0139] Example 3: Comparison of semi-supervised mutation clustering machine learning model with supervised machine learning model
[0140] An exemplary embodiment of the method disclosed herein (eg, SSCM-Pathogenic) is compared to a supervised machine learning model. The supervised machine learning model uses the same features as the exemplary model, but the supervised machine learning model is used (obtained from 1000G (n = 20, 133)) labeled benign gene sequence variation training dataset and (obtained from HGMD (n = 63,363) ) marked pathogenic gene sequence variation training data set for training. In contrast, an exemplary machine learning model (SSCM-Pathogenic) was developed using a labeled training dataset of benign sequence variants and a mixed dataset of unlabeled sequence variants including benign and pathogenic sequence variants trained.
[0141] To test supervised machine learning models and an exemplary model (SSCM-Pathogenic), these models were tested using the ...
PUM
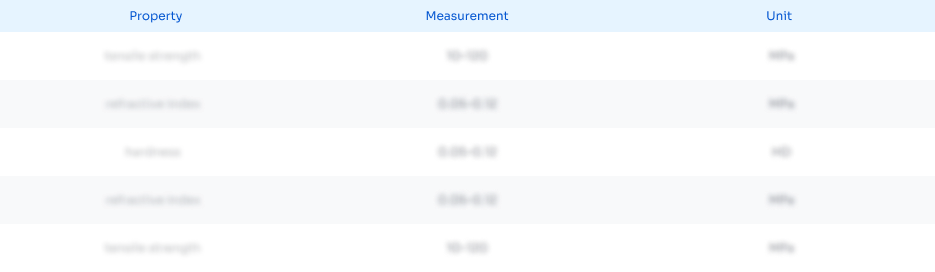
Abstract
Description
Claims
Application Information
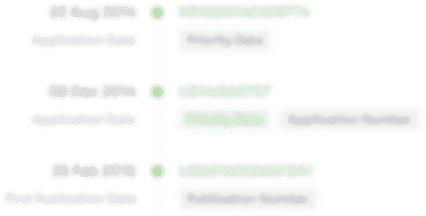
- R&D Engineer
- R&D Manager
- IP Professional
- Industry Leading Data Capabilities
- Powerful AI technology
- Patent DNA Extraction
Browse by: Latest US Patents, China's latest patents, Technical Efficacy Thesaurus, Application Domain, Technology Topic, Popular Technical Reports.
© 2024 PatSnap. All rights reserved.Legal|Privacy policy|Modern Slavery Act Transparency Statement|Sitemap|About US| Contact US: help@patsnap.com