Coal mine water burst predicting method based on long-short-time memory neural network
A technology of long and short-term memory and prediction method, which is applied in special data processing applications, instruments, electrical digital data processing and other directions, and can solve problems such as inability to learn dynamic water inrush data.
- Summary
- Abstract
- Description
- Claims
- Application Information
AI Technical Summary
Problems solved by technology
Method used
Image
Examples
Embodiment
[0066] First, preprocess the original data obtained from the mining area, and use the feature selection method based on the Wrapper evaluation strategy to perform dimensionality reduction operations on the original data set. The steps are as follows:
[0067] Step 1: Obtain the original data according to the analysis results of the water inrush mechanism. The obtained original data includes 14-dimensional water inrush features and 1-dimensional actual water inrush results. The 14 dimensions of the water inrush feature are aquifer thickness, water pressure, distance from the working face, sandstone section thickness, mudstone section thickness, limestone section thickness, coal thickness, coal seam dip angle, presence or absence of structures, fault drop, and fracture zones , mining area, mining height and strike length. All water inrush feature data constitute a p-dimensional water inrush feature set, p=14. All the actual water inrush results constitute a one-dimensional actu...
PUM
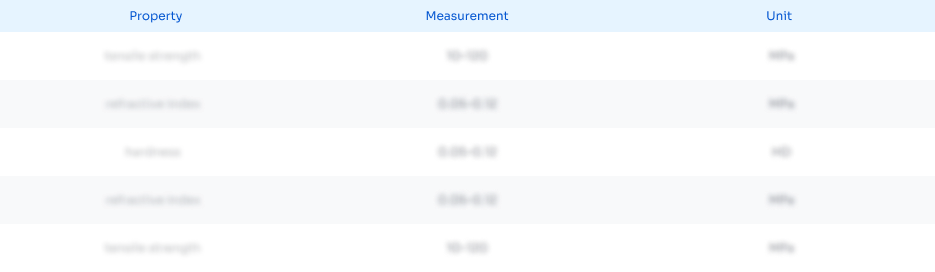
Abstract
Description
Claims
Application Information
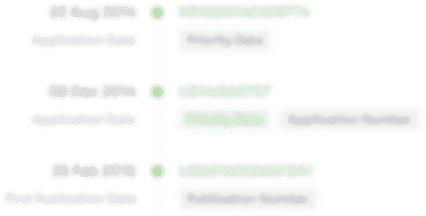
- R&D Engineer
- R&D Manager
- IP Professional
- Industry Leading Data Capabilities
- Powerful AI technology
- Patent DNA Extraction
Browse by: Latest US Patents, China's latest patents, Technical Efficacy Thesaurus, Application Domain, Technology Topic.
© 2024 PatSnap. All rights reserved.Legal|Privacy policy|Modern Slavery Act Transparency Statement|Sitemap