High spectral abnormity detecting method based on dynamic weight deep self-coding
A dynamic weight and anomaly detection technology, applied in the field of hyperspectral anomaly detection, can solve the problems of local model pollution and low detection accuracy, and achieve the effect of solving pollution and improving detection accuracy.
- Summary
- Abstract
- Description
- Claims
- Application Information
AI Technical Summary
Problems solved by technology
Method used
Image
Examples
specific Embodiment approach 1
[0030] Specific implementation mode one: combine figure 1 To illustrate this embodiment, the specific process of a hyperspectral anomaly detection method based on dynamic weight depth self-encoding in this embodiment is as follows:
[0031] Step 1. Input the original hyperspectral image data into the DBN model, train the parameters of the DBN model, and obtain the optimized DBN model;
[0032] Step 2, input the tested image into the optimized DBN model, encode the tested image, and obtain the coded image of the tested image and the corresponding reconstruction error image;
[0033] The measured image is an original hyperspectral image;
[0034] Step 3: Input the coded image obtained in step 2 into the local pixel coding selection block, take a pixel in the measured image as the measured pixel, and obtain the local coded image of the measured pixel for the measured pixel; perform step 5 ;
[0035] Step 4. Input the reconstruction error image obtained in step 2 to the local ...
specific Embodiment approach 2
[0040] Specific embodiment two: the difference between this embodiment and specific embodiment one is: in the step one, the original hyperspectral image data is input into the DBN model, the DBN model parameters are trained, and the optimized DBN model is obtained; the specific process is:
[0041] Step 11, constructing the DBN model, pre-training the DBN model, and obtaining the preliminary estimated value of the DBN model parameters;
[0042] The specific process is:
[0043] The DBN model (DBN neural network) is composed of a multi-layer RBM model, and the single-layer RBM model is as follows: figure 2 shown. Including n visible layers, m hidden layers, and the weight coefficient w connecting visible layers and hidden layers;
[0044] The visible layer v is the input of the RBM, which is a column vector of n×1, and each component corresponds to each spectral band of the hyperspectral image;
[0045] The hidden layer h is the output of RBM, which is a column vector of m×...
specific Embodiment approach 3
[0094] Specific embodiment three: the difference between this embodiment and specific embodiment one or two is that: in the step two, the tested image is input into the optimized DBN model, the tested image is encoded, and the coded image of the tested image is obtained and the corresponding reconstruction error image; the specific process is:
[0095] DBN Inference Image Encoding
[0096] It mainly obtains the image coding after the image passes through the DBN network. The original hyperspectral image is input to the DBN inference coding module, which is a standard DBN model, and its parameters are generated during the training phase of the DBN model. The model structure is as image 3 shown
[0097] Input the tested image to the optimized DBN model. The number of neurons in the middle layer of the DBN model is generally lower than the number of nodes in the input and output layers. The entire DBN model has a symmetrical structure, and the output of the neurons in the mid...
PUM
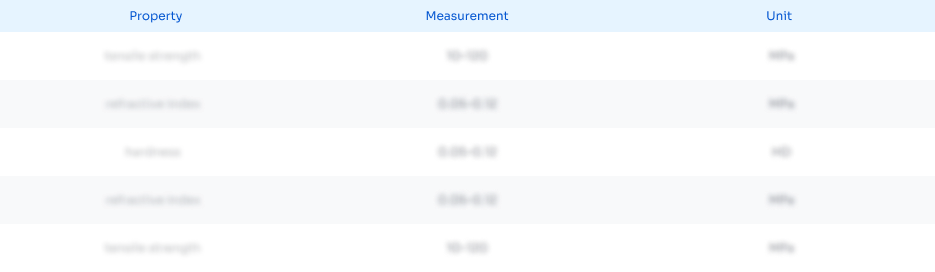
Abstract
Description
Claims
Application Information
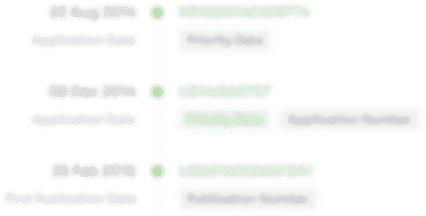
- R&D Engineer
- R&D Manager
- IP Professional
- Industry Leading Data Capabilities
- Powerful AI technology
- Patent DNA Extraction
Browse by: Latest US Patents, China's latest patents, Technical Efficacy Thesaurus, Application Domain, Technology Topic, Popular Technical Reports.
© 2024 PatSnap. All rights reserved.Legal|Privacy policy|Modern Slavery Act Transparency Statement|Sitemap|About US| Contact US: help@patsnap.com