Liver cancer image automatic segmentation method based on random forest and fuzzy clustering
A random forest and fuzzy clustering technology, applied in the field of medical image processing, can solve the problems of limited clinical application range, affecting the recognition effect, time-consuming and labor-consuming, etc., achieving fast calculation speed, good denoising effect, and wide application range Effect
- Summary
- Abstract
- Description
- Claims
- Application Information
AI Technical Summary
Problems solved by technology
Method used
Image
Examples
Embodiment Construction
[0016] The present invention will be further described below in conjunction with accompanying drawing.
[0017] combine figure 1 , input a liver CT image with a size of 512×512×120, denoising by the following variational model
[0018]
[0019] in represents a 2D image area, Represents the current denoised image, and treats the image as a three-dimensional surface, and represent the two principal curvatures of the surface, respectively, represents the Gaussian curvature. The model can be solved by the region decomposition method, and the denoising of the image can be realized after 15 iterations. The original image and the image after denoising are as follows: figure 2 .
[0020] According to the gold standard of images in the training set (segmentation results of liver and tumor), the original images can be divided into three categories: liver, tumor and background. The grayscale features and texture features of the three types of images are extracted respectiv...
PUM
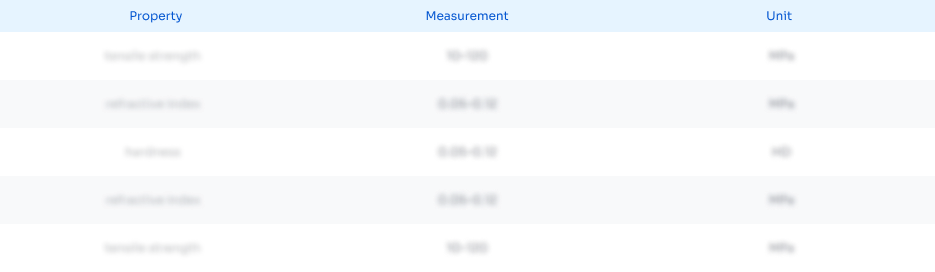
Abstract
Description
Claims
Application Information
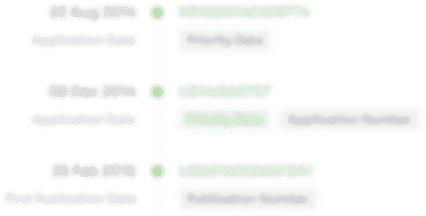
- R&D Engineer
- R&D Manager
- IP Professional
- Industry Leading Data Capabilities
- Powerful AI technology
- Patent DNA Extraction
Browse by: Latest US Patents, China's latest patents, Technical Efficacy Thesaurus, Application Domain, Technology Topic, Popular Technical Reports.
© 2024 PatSnap. All rights reserved.Legal|Privacy policy|Modern Slavery Act Transparency Statement|Sitemap|About US| Contact US: help@patsnap.com