Depth shape priori extraction method
An extraction method and depth technology, which are applied in image data processing, instrumentation, computing, etc., can solve the problems of not establishing a saliency target, not capturing the shape of the depth map, etc., and achieve the effect of expressing information in depth and suppressing background interference.
- Summary
- Abstract
- Description
- Claims
- Application Information
AI Technical Summary
Problems solved by technology
Method used
Image
Examples
Embodiment 1
[0031] A deep shape prior extraction method, see figure 1 , the deep shape prior extraction method includes the following steps:
[0032] 101: Select K superpixel regions with larger RGB saliency values as root seed points, and establish the relationship between depth characteristics and saliency;
[0033] 102: Based on the depth smoothness and consistency constraints, determine the child node set of each root seed point, so as to describe the depth shape attribute;
[0034] 103: Considering the depth consistency of the relevant superpixel nodes in the two rounds of cyclic propagation, and the depth consistency between the current cyclic superpixel and the root seed point, the final DSP value is defined as the maximum depth consistency of the two cases value;
[0035] 104: The final DSP result is obtained after the DSP images generated by multiple root seed points are fused.
[0036] Wherein, before step 101, the depth shape prior extraction method also includes:
[0037...
Embodiment 2
[0046] The scheme in embodiment 1 is further introduced below in conjunction with specific calculation formulas and examples, see the following description for details:
[0047] 201: image preprocessing;
[0048] Let the RGB color image be marked as I, and the corresponding depth map be marked as D. First, the color image is segmented using the SLIC (Simple Linear Iterative Clustering) superpixel segmentation algorithm to obtain N superpixel regions, denoted as Among them, r m is the superpixel region.
[0049] Then, choose an existing RGB saliency detection algorithm as the basic algorithm, such as the BSCA algorithm (saliency detection algorithm based on cellular automata), and obtain the RGB saliency result of each superpixel region, denoting the superpixel region r m The RGB significance value of S i (r m ).
[0050] It has been observed that depth maps usually have the following characteristics:
[0051] 1) Compared with background regions, salient objects tend to...
Embodiment 3
[0082] Combined with the following specific experiments, figure 2 The scheme in embodiment 1 and 2 is carried out feasibility verification, see the following description for details:
[0083] figure 2 A visualization of the deep shape prior descriptor is given. The first column is the original RGB color image, the second column is the original depth map, and the third column is the DSP operator visualization result. It can be seen from Figure 2 that the depth shape description operator proposed by this method can effectively capture the shape information of the salient target in the depth map. The target boundary area is clear and sharp, the internal area of the target is uniform, and the background suppression ability is strong. Depth map description capability.
[0084] Those skilled in the art can understand that the accompanying drawing is only a schematic diagram of a preferred embodiment, and the serial numbers of the above-mentioned embodiments of the present inv...
PUM
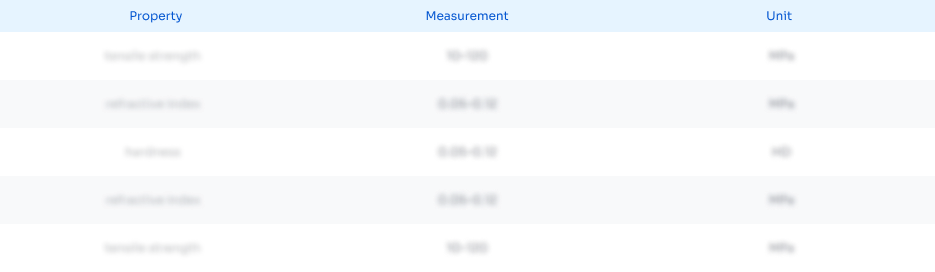
Abstract
Description
Claims
Application Information
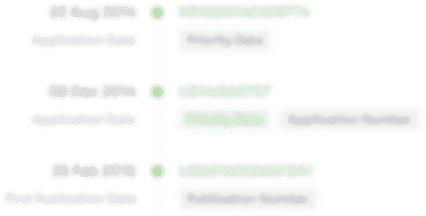
- R&D
- Intellectual Property
- Life Sciences
- Materials
- Tech Scout
- Unparalleled Data Quality
- Higher Quality Content
- 60% Fewer Hallucinations
Browse by: Latest US Patents, China's latest patents, Technical Efficacy Thesaurus, Application Domain, Technology Topic, Popular Technical Reports.
© 2025 PatSnap. All rights reserved.Legal|Privacy policy|Modern Slavery Act Transparency Statement|Sitemap|About US| Contact US: help@patsnap.com