Deep-learning-based multi-mode medical image non-rigid registration method and system
A non-rigid registration, medical image technology, applied in the field of non-rigid multi-modal medical image registration, can solve the problem of low registration accuracy of non-rigid multi-modal medical images
- Summary
- Abstract
- Description
- Claims
- Application Information
AI Technical Summary
Problems solved by technology
Method used
Image
Examples
Embodiment 1
[0090] Step 1 trains the PCANet network. Input N medical images For each pixel of each image, take k without interval 1 ×k 2 The block; vectorize the obtained block and perform de-average. Combining all the resulting vectors together will result in a matrix. Calculate the eigenvector of this matrix, and sort the eigenvalues from large to small, and take the first L 1 The eigenvectors corresponding to the eigenvalues. Will L 1 The eigenvectors are matrixed, and the L of the first layer will be obtained 1 convolution template. Convolving the convolution template with the input image will result in NL 1 images. will this NL 1 The image is input into the second layer PCANet, according to the processing method of the first layer, we will get the L of the second layer PCANet 2 Convolution templates, and get NL 1 L 2 images.
[0091] Step 2 Obtain a PCANet-based structural representation (PCANet-based structural representation, PSR for short) according to the PCANet ...
PUM
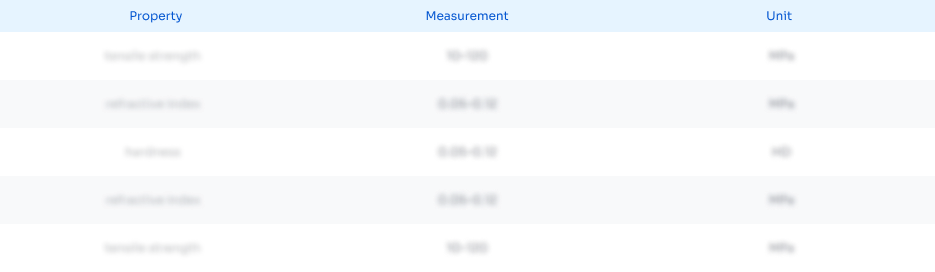
Abstract
Description
Claims
Application Information
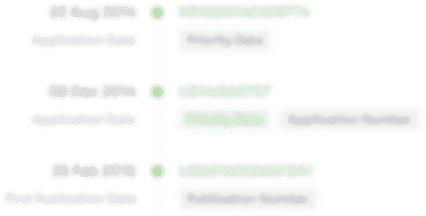
- R&D Engineer
- R&D Manager
- IP Professional
- Industry Leading Data Capabilities
- Powerful AI technology
- Patent DNA Extraction
Browse by: Latest US Patents, China's latest patents, Technical Efficacy Thesaurus, Application Domain, Technology Topic, Popular Technical Reports.
© 2024 PatSnap. All rights reserved.Legal|Privacy policy|Modern Slavery Act Transparency Statement|Sitemap|About US| Contact US: help@patsnap.com