3D Model Classification and Retrieval Method Based on Panorama and Multi-Channel CNN
A technology of three-dimensional models and panoramas, applied in biological neural network models, digital data information retrieval, instruments, etc., can solve problems affecting classification and search accuracy, and achieve the effect of improving robustness
- Summary
- Abstract
- Description
- Claims
- Application Information
AI Technical Summary
Problems solved by technology
Method used
Image
Examples
Embodiment 1
[0032] To solve the above problems, you need to extract multi-resolution panoramic views from each 3D model. Studies have shown that the panoramic view of the 3D model can convert the structure information of the 3D model to 2D image information. [13] . Embodiments of the present invention proposes a three-dimensional model view extraction method based on panoramic map and multi-channel CNN, see figure 1 and figure 2 See the description below for details:
[0033] 101: By passing figure 2 The 3D model surface projected onto the side of the cylinder of R, height H = 2R, figure 2 The 3D model origin is center, the axis of the 3D model is parallel to one of the X, Y, and Z spindle of the space to obtain the initial panorama;
[0034] Where the R value is set to 3 * D max , D max It is the maximum distance of the 3D model surface distance.
[0035] 102: Suppose the Z-axis panorama, the embodiment of the present invention uses a set of points To parameterize the initial panorama;
[0...
Embodiment 2
[0042] The following combines the specific network structure, calculating the formula, image 3 , Figure 4 The training of multi-channel and multi-scale CNN networks and the similarity metrics between two different 3D models are introduced, see the description below:
[0043] image 3 The multi-scale network is displayed. This network includes three scales that extract different resolutions of the same input image, assume the input image size of 256 * 256, for the first scale, the image size is the same as the original picture The size is 256 * 256, and the convolutional neural network directly passes through the VGG16 is characterized by normalization, and the 4096-dimensional mapping is obtained.
[0044] For the second scale, the input image conversion scale becomes 128 * 128, so that the generated picture resolution is 1 / 2 of the original figure resolution, then the sample is sampled, and the convolutional neural network obtains a characteristic map of low resolution pictures. ...
Embodiment 3
[0054] Next, the specific data set, Table 1, Table 2 results in the results of the schemes in Examples 1 and 2, as described below with reference to the following description:
[0055] The data set for evaluating the proposed classification method is the Plinston ModelNet large 3DCAD model data set. ModelNet consists of 127,915 CAD models, divided into 662 object categories, divided into two subsets, ModelNet-10 and ModelNet-40, which contains training and test partitions.
[0056] 1) The ModelNet10 consists of 4,899 CAD models, divided into 10 categories. For convenience of processing, these models are adjusted, and the centroid of the model is placed at the origin of the coordinate, and normalized treatment is performed in translation and rotation.
[0057] Among them, the training and test subsets of ModelNet10 consist of 3991 and 908 models, respectively.
[0058] 2) ModelNet-40 includes 12,311 CAD models, divided into 40 categories. For convenience, these models are adjusted,...
PUM
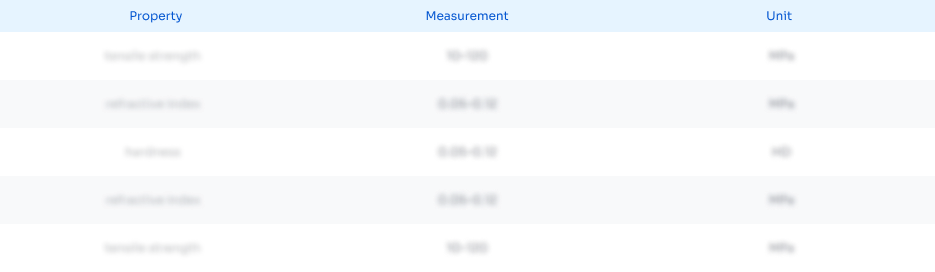
Abstract
Description
Claims
Application Information
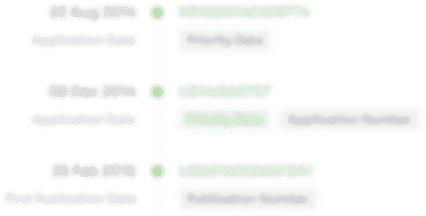
- Generate Ideas
- Intellectual Property
- Life Sciences
- Materials
- Tech Scout
- Unparalleled Data Quality
- Higher Quality Content
- 60% Fewer Hallucinations
Browse by: Latest US Patents, China's latest patents, Technical Efficacy Thesaurus, Application Domain, Technology Topic, Popular Technical Reports.
© 2025 PatSnap. All rights reserved.Legal|Privacy policy|Modern Slavery Act Transparency Statement|Sitemap|About US| Contact US: help@patsnap.com