A significant mining method for characteristic variables of nonlinear systems based on data stream processing
A technology for nonlinear systems and characteristic variables, which is applied in the field of significance mining methods for characteristic variables of nonlinear systems, can solve tedious problems such as neural networks, unrealistic influence of system output, and inability to reduce the dimensionality of nonlinear systems, etc., to achieve The effect of real-time monitoring
- Summary
- Abstract
- Description
- Claims
- Application Information
AI Technical Summary
Problems solved by technology
Method used
Image
Examples
Embodiment Construction
[0017] In order to make the purpose, technical solution and advantages of the present invention clear, the specific implementation manners of the present invention will be clearly and completely described below.
[0018] Such as figure 1 As shown, the flow chart of the method for mining the significance of nonlinear system characteristic variables based on data flow processing in the embodiment of the present application.
[0019] The method includes: step S1: specifying the characteristic variable set and the response variable set in the nonlinear system, collecting massive historical data of each variable in the system, and storing it in the database through data persistence technology after data preprocessing is completed; Step S2: Initialize the two parameters of Eps and MinPts, then perform DBSCAN cluster analysis on the data stored in the database after data preprocessing in the step S1, and classify the data set; Based on the data set after class division, the nonlinea...
PUM
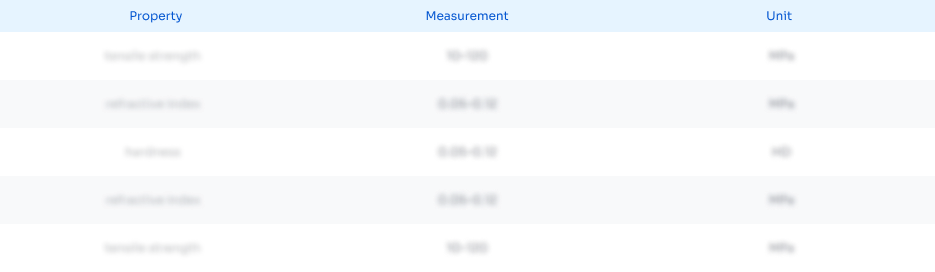
Abstract
Description
Claims
Application Information
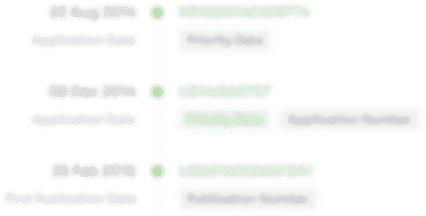
- R&D Engineer
- R&D Manager
- IP Professional
- Industry Leading Data Capabilities
- Powerful AI technology
- Patent DNA Extraction
Browse by: Latest US Patents, China's latest patents, Technical Efficacy Thesaurus, Application Domain, Technology Topic, Popular Technical Reports.
© 2024 PatSnap. All rights reserved.Legal|Privacy policy|Modern Slavery Act Transparency Statement|Sitemap|About US| Contact US: help@patsnap.com